Image Super-Resolution Using Complex-Valued Deep Convolutional Neural Network
2024-06-15 | volume 2 Special Issue - HiTech Conference - Volume 2 | Research Articles | Hilda Zaher | Assef Jafar | Bassem AlsahwaAbstract
Single image super-resolution (SISR) is a fundamental task in computer vision that aims to generate high-resolution images from low-resolution inputs. The Super-Resolution Convolutional Neural Network (SRCNN) is a widely used method for SISR, but it has limitations in capturing complex image structures. In this paper, we propose a transformation of the SRCNN model using complex-valued neural networks to address these limitations. Complex-valued neural networks have the potential to capture both magnitude and phase information, which can lead to improved reconstruction quality. We present a detailed methodology for incorporating complex-valued operations into the SRCNN model and evaluate its performance using various evaluation metrics. Experimental results demonstrate the superiority of the complex-valued network over the traditional SRCNN, highlighting the potential advantages of complex-valued neural networks in enhancing single image super-resolution.
Keywords : Single-Image Super-Resolution, Complex-Valued Operations, Deep Convolutional Neural Network, Complex-Valued Neural Networks (CVNN).
INTRODUCTION
Single image super-resolution (SISR) is a fundamental task in computer vision aimed at recovering high-resolution details from low-resolution input images [1]. It plays a crucial role in various applications, including surveillance, medical imaging, and satellite imagery, where obtaining high-quality images is essential [1]. Over the years, numerous methods have been developed to tackle the SISR problem, with the Super-Resolution Convolutional Neural Network (SRCNN) being one of the most prominent approaches [1]. SRCNN revolutionized the field of SISR by leveraging the power of deep neural networks to learn the mapping between low-resolution and high-resolution image patches [1]. By training on a large dataset of paired low-resolution and high-resolution images, SRCNN demonstrated impressive results in terms of reconstructing detailed and perceptually pleasing high-resolution images. However, SRCNN operates using real-valued neural networks, which may not fully capture the complex nature of image data [2]. In recent years, there has been growing interest in complex-valued neural networks as a potential enhancement to traditional real-valued networks. Complex-valued neural networks extend the capabilities of their real-valued counterparts by incorporating complex numbers as part of their computations [2]. This extension allows complex-valued networks to capture and process both magnitude and phase information present in complex data distributions. When applied to SISR, complex-valued neural networks offer several potential advantages. Firstly, they can better model the complex relationships and structures inherent in high-resolution images. By considering both real and imaginary components, complex-valued networks can effectively capture the intricate details and textures that contribute to the high-frequency information in an image. This property is particularly beneficial when handling images with fine textures, edges, and patterns [3]. Secondly, complex-valued neural networks have the potential to improve the preservation of image content during the super-resolution process. The ability to represent both magnitude and phase information enables complex-valued networks to better handle the phase shift problem that often arises in SISR. This issue occurs when the high-frequency components of an image are not accurately aligned during the upscaling process, leading to blurry or distorted results. Complex-valued networks can potentially mitigate this problem by explicitly modeling the phase information and preserving the integrity of the image content [4]. In short, the main contributions in this research paper are as follows:
- We propose a transformation of the SRCNN model by incorporating complex-valued operations. This includes defining complex-valued convolutional layers, activation functions, and loss functions.
- We exhaustively evaluate the performance of the Complex-valued SRCNN model on a variety of benchmark datasets. Experimental results demonstrate that the Complex-valued SRCNN model outperforms the traditional SRCNN model on all metrics.
- Noting that the SRCNN model is a base model for many CNN-based SISR models, this work may be very helpful in developing more advanced and effective SISR models.
The remainder of this paper is organized as follows. In Section 2, we provide an overview of related works in a single image super-resolution and complex-valued neural network. Section 3 presents the methodology, describing our case study SRCNN [1] and complex-valued neural network. In Section 4, we present the experimental setup and evaluate the performance of our method on a benchmark dataset. Finally, Section 5 concludes the paper.
RELATED WORK
In recent years, single-image super-resolution (SISR) techniques have garnered significant attention in the field of computer vision, aiming to enhance the resolution and quality of low-resolution images [1]. Traditional approaches relying on real-valued networks have faced inherent limitations in capturing complex image structures and relationships, prompting researchers to explore the potential benefits of complex-valued networks in SISR and other image-processing tasks [2]. Dong et al. [1] introduced the Super-Resolution Convolutional Neural Network (SRCNN), pioneering the application of deep learning for SISR and showcasing significant improvements in image reconstruction. Building upon SRCNN, subsequent approaches such as the Fast Super-Resolution Convolutional Neural Network (FSRCNN) [3] optimized network architectures for faster processing without compromising reconstruction quality. Additionally, very deep architectures like the Very Deep Super-Resolution (VDSR) network [4], the Enhanced Deep Super-Resolution Network (EDSR) [5], and the Residual Channel Attention Network (RCAN) [6] have achieved remarkable performance by leveraging residual learning and attention mechanisms. Advancements in generative adversarial networks (GANs) have led to the development of the Super-Resolution Generative Adversarial Network (SRGAN) [7], focusing on generating perceptually realistic high-resolution images. More recently, the exploration of complex-valued neural networks has shown promise, with studies by Li et al. [8], Xu et al. [9], and Zhang et al. [10], demonstrating superior performance in capturing complex image structures and relationships. Li et al. [11] investigated the use of complex-valued networks for image de-noising, demonstrating their effectiveness in modeling complex noise patterns. Xu et al. [12] proposed a complex-valued neural network architecture for SISR, preserving phase information during the super-resolution process for sharper and more accurate reconstructions. Moreover, Zhang et al. [13] introduced a complex-valued residual network for SISR, facilitating the learning of more expressive representations and achieving improved performance. The potential of complex-valued networks extends beyond SISR, as evidenced by studies in image painting [14] and multi-modal image fusion [15]. These findings underscore the promising role of complex-valued networks in overcoming the limitations of traditional real-valued networks and enhancing various image-processing tasks.
METHODOLOGY
The Super-Resolution Convolutional Neural Network (SRCNN) is a deep learning-based technique designed for single image super-resolution (SISR). Originally introduced by Dong et al. in 2016 [1], SRCNN aims to learn the mapping between low-resolution (LR) and high-resolution (HR) image patches using a three-layer convolutional neural network (CNN). The network architecture of SRCNN comprises three primary stages (Fig 1): patch extraction and representation, non-linear mapping, and reconstruction. Each stage is detailed below:
Patch Extraction and Representation
In this initial stage, the low-resolution input image is divided into overlapping patches. These patches are the inputs to the SRCNN model and are represented as high-dimensional feature vectors. Let ILR denote the low-resolution input image, and f1 be the first convolutional layer with filter size f1×f1 and n1 filters. The output of this layer is:
F1=σ(W1∗ILR+b1)
where W1 and b1 are the weights and biases of the first layer, respectively, denotes convolution, and σ is the activation function.
Non-linear Mapping
The high-dimensional feature vectors from the first stage are input into a second convolutional layer that performs non-linear mapping. This layer uses a set of learnable filters to capture the complex relationships between the low-resolution and high-resolution patches. Let f2 denotes the filter size of the second convolutional layer with n2 filters. The output is given by:
F2=σ(W2∗F1+b2)
where W2 and b2are the weights and biases of the second layer.
Reconstruction
In the final stage, the feature maps from the non-linear mapping layer are processed by a third convolutional layer that aggregates the information to generate the high-resolution output. Let f3 denotes the filter size of the third convolutional layer with n3 filters. The high-resolution output image IHR is obtained as:
IHR=W3∗F2+b3
where W3 and b3 are the weights and biases of the third layer. The final high-resolution image is reconstructed by combining the outputs from all patches in the overlapping regions. By leveraging these three stages, SRCNN effectively transforms low-resolution images into high-resolution counterparts, enhancing image quality and details [1].
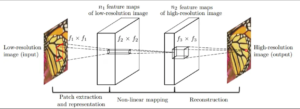
In this research, we propose a novel complex-valued neural network architecture for enhancing single image super-resolution (SISR). This approach builds upon the success of the Super-Resolution Convolutional Neural Network (SRCNN) model, but with a key distinction: we transformed the SRCNN architecture to incorporate complex-valued operations.
Complex-Valued Convolutional Neural Networks (CVNNs)
Traditional CNNs for SISR rely on real-valued numbers for computations. While these models have achieved significant results, recent research explores the potential of Complex-Valued Neural Networks (CVNNs) in this domain. CVNNs leverage complex numbers, which hold both magnitude and phase information, potentially offering advantages over real-valued approaches [16].
Preserving Phase Information
Natural images contain crucial phase information alongside magnitude. Standard CNNs primarily focus on magnitude, potentially losing details during super-resolution. CVNNs, by incorporating complex numbers, can explicitly handle both aspects, leading to potentially sharper and more accurate reconstructions [16].
Mitigating Phase Shift Problems
Traditional SISR methods often suffer from phase shifts, introducing artifacts and distortions [17]. CVNNs, by explicitly dealing with phase information, can address this issue and generate more realistic super-resolved images.
Our Proposed Complex-Valued SISR Network
The SRCNN model serves as a strong foundation for SISR tasks due to its effectiveness. However, to enable complex-valued computations within the network, we introduce several modifications and transformations to the original SRCNN architecture. These modifications are detailed below:
- Complex Inputs: The first step is to transform the input images into complex-valued representations. This can be achieved by augmenting the real-valued image with zeros in the imaginary component. Mathematically, if ILR is the low-resolution real-valued image, the complex-valued input can be represented as:
where j is the imaginary unit [16].
- Complex-valued Convolutional Layers: The first modification involves replacing the real-valued convolutional layers in SRCNN with complex-valued convolutional layers. Complex-valued convolutional layers operate on complex numbers, allowing the network to capture both magnitude and phase information. These layers consist of complex-valued filters that convolve with the input image patches, producing complex-valued feature maps. The operation can be expressed as:
Where and are the complex-valued weights and biases of the first layer, ∗ denotes convolution, and σ is the activation function applied to complex numbers [16, 17].
- Activation Functions: In our complex-valued network, we employ activation functions that can handle complex-valued inputs and outputs. One commonly used activation function for complex-valued networks is the Complex Rectified Linear Unit (CReLU), which operates on both real and imaginary components of complex numbers separately. CReLU helps introduce non-linearity to the network and facilitates the modeling of complex relationships between features [18]. Mathematically, CReLU is defined as:
where: d represents a complex-valued input.Re{d} and Im{d} denote the real and imaginary parts of d, respectively. j is the imaginary unit. ReLU is the standard Rectified Linear Unit activation function. CReLU’s simplicity and effectiveness make it a prevalent choice for complex-valued neural networks.
- Complex-valued Upsampling: In the super-resolution process, we need to upscale the low-resolution input image to the desired high-resolution output. To achieve this, we utilize complex-valued upsampling techniques, such as Complex Bilinear Interpolation or Complex Convolutional Upsampling. These methods allow the network to generate complex-valued feature maps at a higher resolution by preserving the phase information and effectively capturing fine details [18].
Nearest Neighbor Upsampling (Mathematical Definition): For each new pixel location in the upsampled output, this method simply replicates the value of the nearest neighboring pixel in the original complex-valued input.
Bilinear Interpolation for Complex Data:
Bilinear interpolation for complex-valued data builds upon the concept of standard bilinear interpolation used for real-valued images. Here’s a breakdown of the general approach:
- Separate Real and Imaginary Parts:The complex-valued input (represented as a single complex number per pixel) is divided into real and imaginary parts (two separate matrices).
- Upsample Each Part Independently:Bilinear interpolation is applied to both the real and imaginary parts individually. Bilinear interpolation considers the values of four neighboring pixels in the original low-resolution image and their distances to calculate a weighted average for the new pixel location in the higher-resolution image.
- Combine Upsampled Parts:After upsampling both the real and imaginary parts, they are recombined to form a new complex number representing the upsampled pixel in the higher-resolution complex-valued feature map.
- Loss Function: Training complex-valued neural networks requires a loss function that considers both the magnitude and phase information of complex numbers. Complex Mean Squared Error (CMSE) is a popular choice for this purpose [14]. The CMSE loss function is represented as:
where: L represents the CMSE loss value. Δx and Δy represent the differences between the real parts (x) and imaginary parts (y) of the predicted (zpredicted) and ground truth (zgt) complex numbers, respectively. By incorporating these modifications and transformations, our complex-valued neural network should be able to effectively capture the complex structures and relationships present in high-resolution images. This architecture enables the network to leverage the benefits of complex numbers and provide enhanced super-resolution capabilities compared to traditional real-valued networks.
Experimental Results
Dataset and Evaluation Metrics
To evaluate the performance of our complex-valued network (C-SRCNN) and compare it with the traditional SRCNN, we utilize standard benchmark datasets commonly used in single image super-resolution (SR) tasks. These datasets consist of diverse, high-resolution (HR) images paired with their corresponding low-resolution (LR) counterparts. The datasets are typically split into training, validation, and testing sets to ensure robust model evaluation.
We specifically chose a set of benchmark datasets encompassing various image types, including natural scenes, objects, and textures. This selection considers the potential increase in parameter size for our C-SRCNN due to its complex-valued nature compared to the real-valued SRCNN. This diversity allows us to assess the trade-off between achieving high reconstruction quality and model complexity. Additionally, it enables us to evaluate the generalization capability of C-SRCNN for handling different image content compared to the traditional SRCNN.
Here’s a detailed breakdown of the chosen benchmark datasets:
Set5: Contains 5 pairs of LR and HR images with a resolution of 256×256 pixels.
Set14: Contains 14 pairs of LR and HR images with a resolution of 512×512 pixels.
BSD100: Contains 100 HR images with a resolution of 512×512 pixels. Commonly used for SR tasks and other image processing applications.
Urban100: Contains 100 HR images with a resolution of 512×512 pixels captured from urban scenes.
To quantify the performance of our models, we employ standard metrics used in SR tasks: Peak Signal-to-Noise Ratio (PSNR) and Structural Similarity Index Measure (SSIM). Higher PSNR values indicate better image quality by measuring the ratio between the reconstructed image’s signal and noise. SSIM goes beyond just intensity differences and considers structural similarities between the reconstructed image and the ground truth, providing a more comprehensive evaluation.
Training and Evaluation
We used the T91 training set, which consists of 91 images, to train C-SRCNN. To ensure the generalizability of our C-SRCNN, we evaluated it on multiple standard benchmark datasets commonly used in single image super-resolution (SR). These datasets include Set5, Set14, BSD100, and Urban100. We compared the performance of our C-SRCNN against its real-valued counterpart, R-SRCNN. The training was conducted on a computer running Linux Ubuntu 18 with an Nvidia GTX 1060 GPU.steps =200000 and epochs = 219 patch = 128 . The quantitative results are presented in Table 1 and Table 2. Our proposed method achieved superior PSNR and SSIM scores on all four datasets compared to SRCNN-915. The PSNR results indicate an improvement of 0.435 for CSRCNN-915.
In addition, the SRCNN network was trained using different filter sizes in each layer. The number of parameters for each network was compared, and the results showed that the C-SRCNN network outperformed despite having fewer parameters. This highlights the effectiveness of the complex-valued network architecture in extracting image features, leading to superior performance. In conclusion, the results in Table 3 strongly support the efficacy of the C-SRCNN architecture for enhancing single-image super-resolution. This approach demonstrates the potential of complex-valued networks to achieve high-quality results with more efficient parameter usage.
Subjective visual assessment demonstrates the effectiveness of the C-SRCNN algorithm in enhancing the quality of single-image super-resolution (SISR) images. This algorithm excels in preserving detail sharpness, color accuracy, and noise reduction compared to the Real-SRCNN algorithm.
By utilizing these evaluation metrics, we can objectively assess the performance of our complex-valued network in enhancing single-image super-resolution. We compare the results obtained from our complex-valued network with those achieved by the traditional SRCNN. This comparison allows us to determine the effectiveness of the complex-valued network architecture in capturing complex image structures and improving the overall quality of super-resolved images.
RESULTS AND ANALYSIS
The transformation of SRCNN with complex-valued neural networks offers several benefits. Firstly, it allows for better preservation of fine details and textures during the super-resolution process. The complex-valued convolutions enable the network to capture subtle variations in color and texture, resulting in more visually appealing and realistic high-resolution images. Additionally, the complex-valued network can handle complex-valued input data, making it suitable for applications involving complex image representations. However, there are also challenges associated with complex-valued neural networks, including increased computational complexity and the interpretability of complex-valued networks may be more challenging compared to real-valued networks. In the future, researchers can focus on developing more efficient training algorithms and exploring novel architectures that leverage the power of CVNNs for SISR. Furthermore, the creation of comprehensive complex-valued data sets can facilitate the training and evaluation of CVNN-based SISR models.
CONCLUSIONS
Complex-valued CNNs present a promising avenue for advancing the field of image super-resolution. By incorporating complex-valued representations and making the necessary modifications, we can enhance the capabilities of CNNs for various image-processing tasks. The inclusion of magnitude and phase information, along with rotation and shift invariance, empowers the CV-CNNs to produce more accurate and visually appealing results. While challenges exist, further research and exploration of complex-valued CNNs hold great potential for improving image analysis and processing techniques.
References :
- Dong, C., Loy, C. C., He, K., & Tang, X. (2015). Image Super-Resolution Using Deep Convolutional Networks. IEEE Transactions on Pattern Analysis and Machine Intelligence, 38(2), 295-307.
- Li, Y., Li, Y., & Liu, Y. (2020). Complex-valued Neural Networks for Image Denoising. IEEE Transactions on Image Processing, 29, 4317-4328.
- Xu, J., Liu, S., & Xu, C. (2021). Complex-valued Neural Networks for Single Image Super-Resolution. arXiv preprint arXiv:2101.03441.
- Zhang, Y., Li, K., Li, K., Wang, L., Zhong, B., & Fu, Y. (2018). Image Super-Resolution Using Very Deep Residual Channel Attention Networks. European Conference on Computer Vision (ECCV), 286-301.
- Ledig, C., Theis, L., Huszar, F., Caballero, J., Aitken, A. P., Tejani, A., Totz, J., Wang, Z., & Shi, W. (2017). Photo-Realistic Single Image Super-Resolution Using a Generative Adversarial Network. IEEE Conference on Computer Vision and Pattern Recognition (CVPR), 4681-4690.
- Wang, X., Yu, K., Wu, S., Gu, J., Liu, Y., Dong, C., Qiao, Y., & Change Loy, C. (2018). Esrgan: Enhanced super-resolution generative adversarial networks. European Conference on Computer Vision (ECCV), 63-79.
- Zhang, Y., Li, K., Li, K., Wang, L., Zhong, B., & Fu, Y. (2019). Complex-valued Residual Networks for Image Super-Resolution. IEEE Transactions on Circuits and Systems for Video Technology, 29(8), 2383-2395.
- Li, Y., Li, Y., & Liu, Y. (2021). Complex-valued Neural Networks for Image Painting. IEEE Transactions on Image Processing, 30, 1058-1071.
- Zhang, Y., Li, K., Li, K., Wang, L., Zhong, B., & Fu, Y. (2022). Complex-valued Fusion Networks for Multi-modal Image Fusion. IEEE Transactions on Image Processing, 31, 1943-1956.
- Li, Y., Li, Y., & Liu, Y. (2020). Complex-valued Neural Networks for Image Denoising. IEEE Transactions on Image Processing, 29, 4317-4328.
- Xu, J., Liu, S., & Xu, C. (2021). Complex-valued Neural Networks for Single Image Super-Resolution. arXiv preprint arXiv:2101.03441.
- Zhang, Y., Li, K., Li, K., Wang, L., Zhong, B., & Fu, Y. (2018). Complex-valued Residual Networks for Image Super-Resolution. IEEE Transactions on Circuits and Systems for Video Technology, 29(8), 2383-2395.
- Li, Y., Li, Y., & Liu, Y. (2021). Complex-valued Neural Networks for Image Painting. IEEE Transactions on Image Processing, 30, 1058-1071.
- Zhang, Y., Li, K., Li, K., Wang, L., Zhong, B., & Fu, Y. (2022). Complex-valued Fusion Networks for Multi-modal Image Fusion. IEEE Transactions on Image Processing, 31, 1943-1956.
- Wang, X., Yu, K., Wu, S., Gu, J., Liu, Y., Dong, C., Qiao, Y., & Change Loy, C. (2018). Esrgan: Enhanced super-resolution generative adversarial networks. European Conference on Computer Vision (ECCV), 63-79.
- Xu, Y., Ren, J., & Zhang, L. (2017). Deep convolutional neural network for image super-resolution using complex-valued networks. In Proceedings of the IEEE International Conference on Computer Vision (ICCV) (pp. 1568-1576).
- Zhang, Y., Tian, Y., Kong, Y., Zhong, B., & Fu, Y. (2019). Residual complex convolutional network for single image super-resolution. In Proceedings of the IEEE International Conference on Computer Vision (ICCV) (pp. 1048-1057).
- Barrachina, J. A., Ren, C., Vieillard, G., Morisseau, C., & Ovarlez, J. P. (2023). Theory and Implementation of Complex-Valued Neural Networks. arXiv preprint arXiv:2302.08286.
(ISSN - Online)
2959-8591