Using Particle Filter for Estimating Velocity of Maneuvering Target Being Tracked by Passive Radar
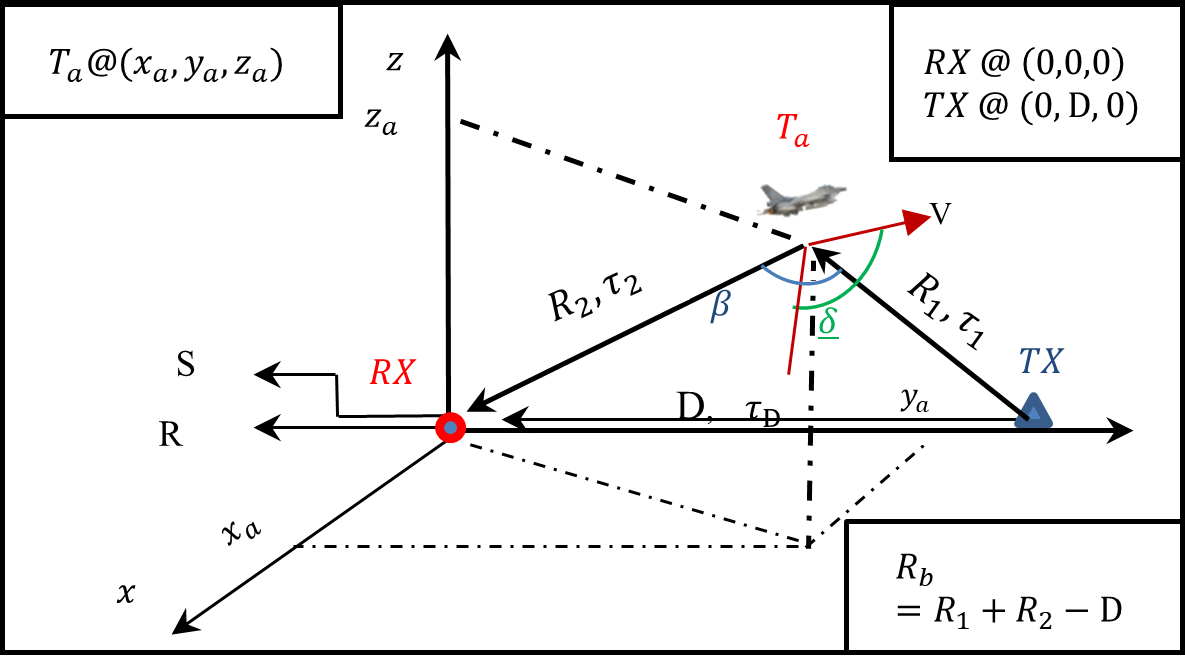
INTRODUCTION
Trans fatty acids (TFAs) are unsaturated fatty acids with at least one double bond that is in the trans configuration. TFA are primarily derived from two sources: (1) ruminant trans fats, which occur naturally in dairy products and meat from ruminant animals; and (2) industrial trans fats, which are generated through the partial hydrogenation of vegetable oils [1]. During the thermal preparation of food, such as frying and baking, small amounts of TFA are also produced [2]. Trans isomers of oleic acid (18:1) are the most common in food products, followed by trans isomers of linoleic acid (18:2 n6), linolenic acid (18:3 n3), and palmitoleic acid (16:1). Cow’s ghee and partially hydrogenated fats have significantly different amounts and qualities of TFA [3]. Conjugated linoleic acid (CLA) is a polyunsaturated fatty acid present in animal fats such as red meat and dairy products [4]. Minimal amounts of CLA are present naturally in plant lipids, and various CLA isomers are generated via the chemical hydrogenation of fats (as illustrated in Figure 1). However, the CLA is not labeled as trans-fats [5].
Over the last three decades, there has been a growing amount of convincing scientific research on the health-damaging consequences of TFA [6]. TFA consumption has been linked to an increased risk of heart disease. TFA may increase the concentration of low-density lipoprotein (LDL) cholesterol while decreasing the concentration of high-density lipoprotein (HDL) cholesterol, both of which are risk factors for coronary heart disease [7]. According to the World Health Organization, TFA consumption should not exceed 1% of total daily energy intake (equal to less than 2.2 g/day in a 2000-calorie diet) [8]. The amount of trans fats in various food products and their daily intake in many countries has been estimated. TFA levels (g/100 g food) ranged from 0 to 0.246 in Argentina [9] and from 0 to 22.96 in India [10]. Ismail et al. [11] evaluated TFA in traditional and commonly consumed Egyptian foods and found that 34% of the products exceeded the TFA limit. Many Countries like Denmark, the United States, and Canada, have begun to reduce and eliminate trans fats in food through legislative initiatives that involved the implementation of regulations setting maximum limits of trans fats or mandated labeling of trans fats [12]. The United Arab Emirates is one of the leading Arab countries that have banned the presence of TFA in food products [13]. To our knowledge, no data on the trans fatty acids (TFAs) content of Syrian foods are available. Therefore, the current work’s objective is to give accurate and up-to-date information on the TFAs content of food products sold in Syria to create a steppingstone for the necessary laws and regulations to impose restrictions on the concentration of TFAs in imported or locally produced foods.
MATERIAL AND METHODS
TFA Standards, Hexan, Methanol, Sodium sulfate, Benzene, and Boron trifluoride were obtained from Merck (Germany). Fatty acids standard, namely: Methyl trans-9-Octadecenoate (elaidic acid methyl ester), Trans-11-Octadecenoic acid methyl ester (trans vaccenic acid methyl ester), Methyl cis-9-hexadecenoate (oleic acid methyl ester), linoleic acid methyl ester mix cis/trans, linolenic acid methyl ester isomer mix cis/trans, were purchased from Sigma-Aldrich (Germany).
Food samples
Seventy-six samples were collected from the local market of Damascus city in 2022. The samples included: Cow’s ghee (n=9), palm oil (n=7), Sardine (n=9), Olive oil (n=30), Soybean oil (n=7), Sunflower Oil (n=7), Flaxseed oil (n=5), and Sesame Oil (n=2).
Extraction of Fat
The Soxhlet method was used to extract the lipids from the samples (except oils) according to the method of AOAC (2019) [14]; briefly, 10 g of the sample were put into extraction thimble, which were extracted using 250 mL of N-Hexane, for 8 h. The extracted fat was used to prepare the methyl esters of fatty acids. The fats’ percentage in sardine samples were determined according to the Soxhlet method, but the fat which used in the preparation of FAME was extracted using a novel “cold method”, briefly, 10 g of the sardine sample was freeze-dried for 8 h. then the freeze-dried material was crushed manually using a porcelain pistol and mortar, transferred into airtight-screw cap glass bottle, soaked in 20 mL of N-Hexane, put in fridge (4°C) for 24 h. The N-Hexane (which containing the fat) was filtered, evaporated with Nitrogen current, and finely used to prepare FAMEs as described above. This novel method protected the polyunsaturated fatty acids (PUFAs), specially EPA and DHA from decomposition.
Fatty acids methyl esters preparation
The methyl esters of fatty acids (FAME) were prepared according to the procedure described by Morrison and Smith [15]. In a test tube, 0.02 g of fat was mixed with 2 ml of high-purity benzene and 2 ml of 7% BF3 in methanol. The tube’s vertical space was filled with nitrogen, closed tightly, and incubated in a boiling water bath (Memmert wb 14 models) for 60 minutes. After the mixture was cooled, 2 ml of n-hexane and 2 ml of water were added, and the tubes were centrifuged at 2000 rpm for 5 min. The supernatant was collected, transferred to a clean tube, mixed with 2 ml of water, and centrifuged again. The hexane layer was separated and mixed with anhydrous sodium sulphate, and 1μl was taken and injected into a gas chromatograph.
GC condition
TFA was determined using a gas chromatograph (Shimadzu 17A, Japan) equipped with a Split/Splitless injector and a flame ionization detector (FID). A capillary column, PRECIX HP 2340 (60 m x 0.25 mm x 0.20 m film thickness), was used to separate and quantify each FAME component. The oven temperature program was set at 175 oC for 18 minutes, then the temperature was increased to 190oC at 5°C per minute, and the final temperature (190 oC) was maintained for 12 minutes. Nitrogen was used as the carrier gas at a flow rate of 1 mL/min. Methyl trans-9-Octadecenoate (elaidic acid methyl ester), Trans-11-Octadecenoic acid methyl ester (trans vaccenic acid methyl ester), Methyl cis-9-hexadecenoate (oleic acid methyl ester), linoleic acid methyl ester mix cis/trans (including CLAs, linoleic acid and trans linoleic acid), linolenic acid methyl ester isomer mix cis/trans, were identified (as shown in figure 1). The quantitative determination of trans fatty acid was calculated according to the peak areas.
RESULT
The results in Table 1 showed that the total TFA in cow’s ghee ranged from 0.63 to 4.78 g/100 g. The results also showed that vaccenic acid was the major trans-18:1 isomer in the samples. Also, CLA with a concentration ranging from 0.21 to 1.35% was detected in cow’s ghee samples (Table 1).
The trans fatty acid content of the olive oil samples investigated is shown in Table 2 and varied between 0.0103 and 0.3349 g/100 g oil.
TFAs in Soybean, Sunflower, Flaxseed, and Sesame oils
The Trans fatty acids content in soybean oil varied between 0.042 and 2.32 g/100g (Table 3). Table 3 shows the overall percentages (%) of TFA isomers detected in the sunflower oil samples tested. The TFA concentrations in the samples ranged from 0.13 to 1.23 g/100g. Table 3 also shows the distribution of TFAs in commercial flaxseed oil samples. The most common TFA isomers found in flaxseed oils were C18:3, followed by C18:2. While C18:1 trans-9, C18:1 trans-11, and CLA were not detected (as shown in Figure 3).
Table 3 illustrates the percentage of TFA in two examined sesame oil samples. The TFA concentrations observed in sesame oil ranged between 0.06 and 0.35 g/100 g (as shown in Figure 4)
TFAs in Sardine
TFA levels in sardine samples ranged from 0.087 to 0.75% (Table 5).
DISCUSSION
Cow’s ghee contains about 2.7% TFA with one or more trans-double bonds. Our results for TFAs in milk are consistent with the findings of Precht and Molkentin [16] and Vargas-Bello Pérez and Garnsworthy [17]. According to Shingfield et al [18], the trans-11 isomer is the main trans fatty acid in the group of trans-C18:1 isomers in cow’s ghee and represents about 40–50% of the total C18:1 trans fatty acids. Natural TFA, like vaccenic acid (18:1 t11), has anti-atherosclerotic and anti-diabetic properties [19]. The difference in vaccenic acid concentration between the samples investigated could be attributed to differences in the animal feeding system, which affects the amount of this isomer in cow’s ghee [20]. Dairy products contain the natural trans fatty acid conjugated linoleic acid (CLA), which has been linked to a lower risk of heart disease [21]. Vargas and Garnsworthy [17] confirmed that rumen bacteria can biohydrogenation unsaturated fatty acids and produce CLA. The variation in CLA concentration between samples is due to the difference in predominant microbial species and the diet offered to the animal [22]. All the olive oil samples studied were within the Syrian National Standard specification of olive oil (C18:1 T ≤0.05) [23], except for two samples for a total of C18:2 T + C18:3 T. This study’s results agree with the findings of Sakar et al. [24], who found that the trans fatty acid level of Moroccan olive oil was between 0.09 and 0.04 g/100 g fat. The total TFA content of Costa Rican and Egyptian olive oil samples is around 0.23 and 0.4 g/100 g, respectively [25] [11]. The amount of trans fatty acids in the Syrian olive oil samples varied, which may be related to differences in olive types, geographic area, and extraction methods. Sakar et al. [24] found a high level of TFA value in the super-pressure system, while the lowest one was displayed by the traditionally extracted oil, and the TFA correlated positively to K232, K270, and acid values. Our data revealed that the mean levels of TFA in soybean oil (1.0056%) were lower than the TFA concentration in Malaysian soybean oil (5.79%) [26]. The most frequent TFA in the soybean oil samples were C18:2 trans, C18:3 trans, and C18:1 trans-9. Soybean oil often contains more C18:2 trans isomers than C18:1 trans fatty acids, according to Aro et al. [27]. TFA in sunflower oils is probably generated by heating sunflower seeds before or during the extraction process [28]. Hou et al. [7] examined 22 samples of sunflower oil and found that the mean TFA was 1.41%, while Hoteit et al. [29] found a low concentration of TFA in sunflower oil samples (<0.1%) collected from the Lebanese market. C18:3 and C18:2 were the most common TFA isomers in flaxseed oils, which could be attributed to the lower levels of oleic and linoleic acids in linseed oil, which have been shown to be more stable than linolenic acid [30]. Bezelgues and Destaillats [31] reported that commercial linseed oil for human consumption is refined; during the refining process, TFA forms due to high amounts of unsaturated fatty acids (UFA) and high temperatures, particularly during the deodorization step. According to Johnson et al. (2009) [32], the total TFA content in Indian sesame oil was 1.3%, and Elaidic acid comprised the majority. Sesame oil’s TFA in the Malaysian market ranged from 0.1 to 0.76%. While Song et al. (2015) [33] did not detect any TFA in Korean sesame oil. The presence of TFA in refined palm oils is due to thermal isomerization caused by the relatively high temperatures of up to 260°C used during deodorization [34]. According to Hishamuddin et al. [6], TFA levels in Malaysian palm oil range between 0.24 and 0.67 g/100g. Wolff [35] has previously demonstrated that TFA formation is strongly influenced by heating time and deodorization temperature; thus, longer deodorization times at higher temperatures may increase the TFA content of refined oils. TFA was also detected at low levels in frozen sardine samples (Table 5). Nasopoulou et al. [36] found the levels of C18:1 trans (ω-9) in raw, grilled, and brined sardines to be 39.7, 65.3, and 96.7 mg/kg fish tissue, respectively.
CONCLUSION
The study’s findings indicated that TFA of natural origin (Vaccenic acid) could be differentiated from TFA of industrial origin (Elaidic acid) in the products analyzed. We found that cow’s ghee contained the highest percentages of CLA, which is known to have health advantages. Olive, sesame, and flaxseed oils are healthy oils that have low levels of TFA. Future studies will focus on the levels of TFA in other food products, especially chocolate bakery products, fast food consumed, and foods common in the Syrian local market.
INTRODUCTION
Bioplastics are biodegradable materials derived from renewable sources and offer a sustainable solution to the problem of plastic waste. Unlike traditional plastics, they are not derived from non-renewable fossil resources such as oil and gas[1]. Bioplastics can be made from a variety of natural materials such as agricultural waste, cellulose, potatoes, corn, and even wood powder. These materials are converted into bioplastics through various preparation processes that exploit existing plastics manufacturing infrastructure to produce bioplastics that are chemically similar to conventional plastics, such as bio polypropylene [2]. A common type of bioplastic is polyhydroxyalkanoate (PHA), a polyester produced by fermenting raw plant materials with bacterial strains such as Pseudomonas. It is then cast into molds for use in various industrial applications, including automotive parts. Bioplastics have many advantages such as carbon footprint, which is defined as the total amount of greenhouse gases (GHGs), specifically carbon dioxide (CO2) and methane (CH4), emitted directly or indirectly by an individual, organization, event, or product throughout its life cycle. This measurement is typically expressed in equivalent tons of CO2 reduction, energy saving in production, avoiding harmful additives such as phthalate or bisphenol A; being 100% biodegradable, bioplastics have applications in sectors such as medicine, food packaging, toys, fashion, and decomposable bags [3][4]. Bioplastics are characterized by their environmentally friendly nature and their ability to reduce plastic pollution. While they offer a promising alternative to traditional plastics, there are challenges related to production and land use costs, energy consumption, water use, recyclability, etc. Ongoing research aims to develop more environmentally friendly types of bioplastics and improve production processes for a more sustainable future, especially at the domestic level and not just at the industrial level [5].
Comparison of bioplastics with traditional plastics in terms of cost and durability
Bioplastics have a lower carbon footprint than synthetic plastics, help in the reduction of CO2 emissions, save fossil fuels, and eliminate non-biodegradable plastic waste. [6] [7] Conversely, traditional petroleum-based plastics from non-renewable resources have a higher environmental impact and do not biodegrade. This petroleum based plastics contribute significantly to pollution that persists in the environment for hundreds of years, causing pollution and damage to ecosystems. While traditional plastics are durable, low-cost, and waterproof, they face challenges due to their slow degradation and negative environmental impacts, especially on marine life where most plastic waste resides [6]. When comparing cost and durability, bioplastics may face production cost challenges compared to conventional plastics, due to factors such as the industrial refining of polymers from agricultural waste [8]. In terms of cost and mechanical durability, the production of bioplastics is more expensive than the production of conventional plastics, which is one of the challenges facing the production of bioplastics at the local and even global level. For example, their production from agricultural waste requires additional costs due to the necessary and multiple stages of refining and isolating the industrial polymers to be used in production. In addition, not all bioplastics have the same durability, thermal stability, and waterproofing properties as conventional plastics. However, many current researches are focused on developing bioplastics that can compete with traditional petroleum-based plastics.
History of the bioplastics industry
The bioplastics industry dates back to the early 20th century when companies began producing bioplastics as an alternative to traditional petrochemical-based plastics. [5][9].
The first attempt to produce bioplastics was in 1862 when Alexander Parkes produced the first man-made plastic called Parkesine. It was a biological material derived from cellulose that, once prepared and molded, maintained its shape as it cooled [10] [11] [9].
Production Methods
Bioplastics are made using various processes, including the conversion of natural materials into polymers suitable for commercial use.
These processes include microbial interactions and nanotechnology synthesis methods, such as crystal growth and polymer extraction from microbes. Using raw materials such as cornstarch, sugar cane, vegetable oils, and wood powder are common in bioplastics production [12].
Current challenges and prospects
The environmental benefits of bioplastics offer advantages such as reducing the carbon footprint, saving energy in production, and avoiding harmful additives found in conventional plastics [13]. Bioplastics represent a promising alternative to traditional plastics due to their lower environmental impact; however, their production costs remain higher, which limits their market adoption. Recent research is focused on improving production efficiency and reducing costs through innovative technologies and sustainable practices. These efforts include utilizing microbial processes, enhancing fermentation techniques, and leveraging agricultural waste as raw materials. By addressing these economic challenges, bioplastics can become a more viable option, contributing to environmental protection and reducing dependence on fossil fuels. Ultimately, the advancement of bioplastic production will play a crucial role in promoting sustainability and mitigating the adverse effects of plastic pollution[14][15] . Many agree that improving the material properties of bioplastics is important for wider adoption and market competitiveness [16].
Benefits of using bioplastics
Bioplastics offer a multitude of advantages over traditional plastics, positioning them as a more sustainable and eco-friendly alternative to mitigate the carbon footprint associated with plastic manufacturing. Moreover, they help to reduce the consumption of fossil fuels [17]. Bioplastics exhibit accelerated decomposition rates, decomposing within a span of a few months in stark contrast to the centuries required for conventional plastics to degrade[18]. This rapid degradation contributes significantly to mitigating environmental pollution and minimizing the presence of microplastics in ecosystems. Notably, bioplastics are derived from renewable resources, supporting sustainable practices by utilizing annually replenishable materials[11]. Bioplastics are considered toxin-free, because they are derived from natural materials and do not contain harmful or toxic chemicals commonly found in conventional plastics, and they degrade without releasing harmful substances [19]. Bioplastics can be fully recyclable and biodegradable, providing a closed system for maximum sustainability impact, they provide improved waste management solutions by reducing the number of plastics sent to landfills and encouraging recycling practices [20] [21].
MATERIALS AND METHODS
Main chemicals used in bioplastic manufacturing
Animal Gelatin, Acetic Acid, Glycerin, Corn Starch
Animal Gelatin: Food-grade animal gelatin, derived from collagen, [22] was used in this study due to its excellent techno-functional properties, including water binding and emulsification. It can be produced domestically by soaking animal bones in hydrochloric acid to remove minerals, followed by extensive washing to eliminate impurities[23] . The cleaned bones are heated in distilled water at 33°C for several hours, then extracted and placed in water at 39°C for further extraction[24]. The resulting liquid undergoes chemical treatment to produce pure gelatin, which is then concentrated, cooled, cut, and dried to achieve optimal quality and gel strength. [25] [26]
Acetic Acid: Acetic acid is utilized in the production of polyethylene terephthalate (PET) and polyvinyl acetate (PVA). It serves as a solvent in oxidation reactions and enhances the properties of plastics, such as elasticity and transparency. [27]
Glycerin: Glycerin is a non-toxic, water-soluble polyol compound that provides flexibility and mechanical strength in bioplastics while enhancing texture and material stability[28].
Corn Starch: Corn starch, composed of amylose and amylopectin, serves as a carbohydrate reserve for plants[30] [29]. Its extraction involves cleaning the grains (Zea mays saccharata),[31] soaking them in a dilute sulfur dioxide solution at 47°C with a pH of 3.5 for 48 hours, followed by crushing, sedimentation, washing, and drying to obtain powdered starch. [32]
protocol for making bioplastics from starch and animal gelatin
First, 1.5 g of cornstarch was weighed using an accurate balance (or equivalent to 3% of the weight/volume of the final mixture), then 3 g of commercially available animal gelatin powder used in the manufacture of sweets is weighed using an accurate balance (or equivalent to 6% of the weight/volume of the mixture). These chemicals are dissolved in 50 ml of tap water (or the volume that achieves a ratio of 3% cornstarch and 6% animal gelatin) at room temperature and, while stirring by a small magnetic stirrer to ensure complete dissolution of the gelatin and homogeneity of the solution, 1.5 ml of pure glycerin is added, then 1.5 ml of commercial acetic acid is added using a graded cylinder. The mixture was gently stirred until the ingredients were homogeneous, then placed in a microwave oven [33], covered with a thin cloth on top, and turned on for 2 minutes, checking the thickness of the mixture every 30 seconds. The microwave oven is turned off until the boiling foam disappears, then heating is continued until the end of the two minutes. After removing the mixture from the microwave oven, the mixture is cooled with running tap water by letting water stream on the outside of the bowl until the temperature of the bowl reaches 60° C. The resulting mixture is poured directly into a Petri dish or other suitable surface and spread out until its dimensions are homogeneous and its thickness is uniform over the entire surface, preferably 4 mm of height in a regular Petri dish. Finally, the mixture is left at room temperature until it hardens, which can take 16-24 hours or longer depending on the thickness and exposure to higher temperatures. In this study, seven mixtures of bioplastics with different materials were prepared and compared, including: potato starch, corn starch, wheat starch, animal gelatin, plant-based gelatin (agar-agar), animal gelatin with cornstarch, and animal gelatin with plant-based gelatin (agar-agar), if we counted also the solvents and enhancements like adding wax to make the bioplastic waterproof and antibiotic and antifungals to make it resistant to bacteria and mold, there would be 22 different compositions tested in this study, the table of these tests is provided in table s1 in supplementary materials.
RESULTS
The evaluation of the bioplastic mixtures revealed that the optimal formulation was a combination of corn starch and animal gelatin. Upon solidification, this mixture produced a cohesive bioplastic with a flexible texture and sufficient strength, making it suitable for a range of packaging applications. Notably, this bioplastic is capable of decomposing in soil within a period of 3 to 4 months or when immersed in water for approximately 3 weeks. Furthermore, it can retain its functional properties for up to 6 months when stored at room temperature and shielded from moisture.
Moisture content (MC)
The moisture content of the plastic was calculated by comparing the initial weight (W1=2 mg) of the bioplastic film (2 cm × 2 cm) and the final weight (W2=1.5 mg), which was determined after a 10 min oven-dry period at 120° c, as shown in equation 1. The result showed that the moisture content was 25%, and the shape of the results is shown in Fig. 1.
Fig1. A comparative image of a bioplastic sample before and after heating in an oven with visually observed changes.
The density of the bioplastic
The density of the bioplastic film (2 cm × 2 cm) was determined by measuring the mass (M) and area (A) of the known bioplastic film thickness (d) using equation 2, and the density was 0.16 g/cm-3 for the cornstarch and animal gelatin mixture bioplastic.
Density = M/A×d [35]
Density of corn starch and animal gelatin mixture = 12/50.24×1.5= 0.16 g cm−3
Water solubility
The sample of cornstarch and animal gelatin mixture (2 cm x 2 cm), was submerged in 30 ml of water for 48 hours at 27 ° c, and no complete dissolution of the sample was observed, indicating that the bioplastic film did not fully dissolve in the water. It has lost approximately 50% of its total weight. However, the flexibility and tensile strength of the sample were observed to have changed. The bioplastic film, which was initially flexible, became coarse and lost its ability to withstand tensile stress, resembling the properties of non-tensile nylon, in a simple finger touch evaluation. [36] The samples made from cornstarch partially dissolved in water, whereas the sample made from animal gelatin transformed into a gel-like consistency. Meanwhile, the sample composed of both starch and animal gelatin altered its texture to resemble that of nylon. [37] Adding 1g of melted wax to overcome water solubility for longer periods resulted in crumbly textures and heterogeneity due to the incompatibility of starch with wax. However, applying dry wax to the surface by rubbing on the solid bioplastic film showed better results, but the water tolerance was acceptable in terms of time (3 months) without the wax.
Biodegradability
A 4 cm x 2 cm sample of the bioplastic was placed in red soil and exposed to the outdoor environment. The soil was watered once a week for the first three weeks to mimic agricultural water exposure. After this time, it was observed that the sample had lost a small amount of its flexibility. The average temperature during this initial three-week period was 22o c in the morning and 10o c at night. In the sixth week, the soil was watered every 4 days, and the temperature increased to 24o c in the morning and 13o c at night. After 4 weeks of this experiment, it was found that the bioplastic had become brittle and fragile, with no flexibility remaining. Additionally, a noticeable reduction in the thickness of the sample was observed as shown in fig2. [38] To prevent mold, 0.5g of antifungal clotrimazole was added at a concentration of 100 ml of the mixture at 30o c. This showed fragility in texture, non-homogeneity, difficulty in drying the sample, and incoherence, as shown in Fig Image 7.
Comparison of different materials used to make bioplastics
After trying different mixtures and methods of making bioplastics, the following results were observed; The Potato starch mixtures did not give good results as the samples were fragile, brittle, and gave low durability. Corn starch mixtures gave good results in terms of durability and flexibility but didn’t withstand tension and pressure. Wheat starch also showed good results. The resulting plastics are flexible and can withstand light and heavy tension and pressure. Animal gelatin provides a hard plastic texture suitable for packaging but does not withstand moderate tension. Plant gelatin (agar-agar) yielded unsatisfactory results, the resulting plastics are fragile and lack cohesion and do not withstand light pressure. Animal gelatin mixed with cornstarch achieved the best results in terms of durability, consistency of texture and ability to withstand medium to high pressure. The addition of the antifungal clotrimazole (each 1g of product powder contains 10 mg of clotrimazole (1-Orto chloro-benzyl imidazole)) resulted in inconsistent texture and poor outcomes. Adding a layer of antibiotic (ampicillin 500 mg dissolved in 5 ml) by using a cotton spreader created a stiff texture in the bioplastic. A view of most of these results is shown in fig3 as follows: 1) animal gelatin, 2) corn starch, 3) wheat starch, 4) animal gelatin & potato starch, 5) potato starch, 6) animal gelatin & potato starch & wax, 7) corn starch & antifungal , 8) wheat starch & antifungal & wax, 9) corn starch & antibiotic, 10) plant gelatin (agar-agar) & antibiotic, 11) plant gelatin (agar-agar), 12) plant gelatin (agar-agar) & animal gelatin, 13) plant gelatin (agar-agar) & corn starch, 14) corn starch & wax, 15) plant gelatin (agar-agar) & wheat starch.
Transparency
The bioplastic made from animal gelatin and also the mixture of animal gelatin with corn starch showed the best results in transparency, and the transparency was different according to the thickness of the liquid mixture before solidifying. Transparency test is shown in Fig4.
Microscopic structure
Examining the bioplastic made from the mixture of animal gelatin and corn starch under the microscope showed consistent appearance of several groups of units with few borders that were more permeable to light as shown in fig5.
All the properties and results of the different mixtures are summarized in Table 1, which gives an estimated evaluation of each mixture used in the study in general, without additives such as wax and antibiotics and antifungals.
This study aimed to compare the effectiveness of different types of bioplastics made domestically, the properties of bioplastics varied greatly between materials, this can be beneficial in some aspects to use a type that gives a certain property in a desired application. Although wheat starch is cracked, it still has a rubbery and spongy texture that can be tested for shock absorbance and transfer fragile materials. Biofilms that were made from animal gelatin can be tested to be used in making bags.
Moisture Content: This mixture demonstrated a large decrease in moisture content when placed in the oven, losing about 75% of its moisture content. This could render this plastic unsuitable for most applications as the resulting water absorption would alter the properties of the plastic and reduce its tensile strength. Therefore, it is recommended that future studies be conducted to increase the moisture content. [39]
The density of the bioplastic: Plastic density influences the arrangement of molecular chains and intermolecular forces. Higher density indicates more tightly packed molecular chains and stronger intermolecular forces, resulting in greater strength and hardness. In contrast, lower density plastics have looser molecular arrangements and weaker intermolecular forces, enhancing flexibility, impact resistance, and transparency. This mixture demonstrated low density, which makes it suitable for use in packaging and plastic bags.
Biodegradability: The loss of moisture mentioned in the results show that this bioplastic can degrade over time in the absence of humidity, but also the water solubility property can make this plastic also degradable in aquatic environments, also soil exposure in the presence of water indicated by the biodegradability results showed that this bioplastic can be released into the environment and degraded in a matter of months.
Adding antifungal and antibiotic: Adding antifungal and antibiotic to the bioplastic showed that mixing the additive with the bioplastic created a fragile texture, while rubbing the additives on the dry bioplastic gave better results. The transparency of the bioplastic can be obtained by altering the thickness of the film and essentially having animal gelatin in the mixture, and the more you have a higher percentage of animal gelatin the more transparent your biofilm can be. Mixing animal gelatin with corn starch provided several benefits of the separate biomaterials, where cornstarch gave the rubbery structure and animal gelatin gave rigidity and transparency, and this mixture was selected as the best based on its stability and texture, yet more rigorous tests are recommended to further evaluate this bioplastic. The Protocol provided in this study is simple and can be used domestically and upscaled commercially to make bioplastic more available and integrated into daily culture.
CONCLUSIONS AND RECOMMENDATIONS
In this study, several biomaterials were investigated to make bioplastic domestically, to achieve economic efficiency and sustainability. Among the different combinations tested, the most promising results were obtained from the combination of animal gelatin and cornstarch. This particular formulation exhibited properties comparable to petroleum-based plastics, making it a suitable option for several domestic applications. Other mixtures were neglected and not further tested because of their breakage and dissociation. The protocol described in this research can be tested and improved to create even novel mixtures of bioplastics derived from animal gelatin sources such as bones from butchers or slaughterhouses and corn starch from crop residues, we were able to make a few yet to have an abundant quantity for tests we had to buy commercial gelatin. This study is considered initial research, and further rigorous tests of this gelatin-corn starch bioplastic are recommended, such as an FTIR test, mechanical property curves, in-vitro fungal tests to obtain more concrete results. possible implementation for use in greenhouses instead of oil-based plastic for it to be dissolved in the soil and become a fertilizer; with the benefit of protection from bioplastic contamination, or in packaging materials intended for food, where transparency and tamper-resistance are essential, but applications of packaging jewelry or small electronics like headphones or book leathering is very much possible with this mixture, all these applications needs further exploration. Bioplastic manufacturing is very feasible on a domestic approach, and it can reduce the use of petroleum-based plastic if adopted by families and societies whenever it is applicable, therefore we recommend its use and further studies on its application in several fields in packaging and preservation. We also recommend studying other forms of hard bioplastic to manufacture rigid bioplastic alternatives to regular plastic, such as biodegradable forks, knives, plates, etc.
INTRODUCTION
Growth factors assume crucial roles in regulating cell proliferation, growth, and differentiation under both physiological and pathological conditions. One such pivotal factor is the platelet-derived growth factor (PDGF), which participates in various physiological activities. PDGF contributes to the differentiation of embryonic organs, facilitates wound healing processes, regulates interstitial pressure within tissues, and plays a key role in platelet aggregation. The multifaceted involvement of PDGF underscores its significance in maintaining homeostasis and responding to dynamic cellular processes in health and disease (1, 2). PDGF is a dimeric polypeptide, each monomer weighing approximately 30 kDa and consisting of nearly 100 amino acid residues. Five isoforms of PDGF exist, denoted as AA, BB, CC, DD, and AB. These isoforms act as activators of the PDGF receptor (PDGFR), which is present in two isoforms, PDGFR-α and PDGFR-β. The activation process involves receptor homo- or hetero-dimerization, leading to the induction of autophosphorylation on specific tyrosine residues located within the inner side of the receptor. This autophosphorylation event triggers the activation of kinase activity, initiating the phosphorylation of downstream proteins (3). The ensuing phosphorylation cascade orchestrates the effects of the PDGF signaling pathway (4). PDGF is involved in a number of malignant and benign diseases, including glioblastoma multiforme (GBM) (5), meningiomas, chordoma, and ependymoma (6, 7). Additionally, PDGF plays a role in skin cancer, specifically dermatofibrosarcoma protuberans (DFSP) (8), gastrointestinal tumors (GIST), synovial sarcoma, osteosarcoma , hepatocellular carcinoma, and prostate cancer (3, 9). Aberrantly elevated levels of PDGF receptor and/or PDGF have been observed in lymphomas and leukemias, including chronic myelogenous leukemia (CML) (10), acute lymphoblastic leukemia (ALL), chronic eosinophilic leukemia (CEL), and anaplastic large cell lymphoma (11, 12). Moreover, such abnormal upregulation has been noted in other cancer types, such as breast carcinoma, sarcomatoid non-small-cell lung cancer, and colorectal cancer (13). These findings underscore the potential role of dysregulated PDGF signaling in the pathogenesis of these hematologic and solid malignancies, suggesting its relevance as a target for further therapeutic exploration. PDGF exerts its influence not only in malignant diseases, but also in non-malignant conditions, extending its influence to fibrotic diseases such as kidney, liver, cardiac, and lung fibrosis. Additionally, PDGF plays a role in various vascular disorders, including systemic sclerosis, pulmonary arterial hypertension (PAH), endothelial barrier dysfunction, proliferative retinopathy, cerebral vasospasm, and cytomegalovirus infection (14, 15). The broad spectrum of PDGF involvement highlights its significance in the context of diverse pathological processes, emphasizing its potential as a therapeutic target in addressing both malignant and non-malignant disorders. Inhibition of the PDGF signaling pathway holds significant therapeutic potential for both malignant and non-malignant diseases. Various strategies have been devised to impede this pathway, including the utilization of monoclonal antibodies targeting PDGF or PDGFR. These antibodies specifically obstruct the PDGF signaling pathway by binding to PDGF or PDGFR, thereby preventing receptor dimerization (16, 17). Alternatively, small molecule inhibitors of receptor kinases present another strategy, although they may lack specificity and inadvertently inhibit other signaling pathways (18). Another approach involves the use of soluble receptors that compete with PDGFR for binding to the ligand, thereby preventing the interaction between PDGF and its receptor. Furthermore, DNA aptamers, oligonucleotides that bind to PDGF and hinder its interaction with its own receptor, represent an additional avenue for therapeutic intervention (19). These diverse strategies offer a range of options for modulating the PDGF signaling pathway with the aim of treating various diseases. Imatinib, a tyrosine kinase inhibitor that effectively impedes the PDGF pathway, has been approved for the treatment of chronic myelogenous leukemia (CML), acute lymphoblastic leukemia (ALL), chronic eosinophilic leukemia (CEL), gastrointestinal stromal tumors (GIST), and dermatofibrosarcoma protuberans (DFSP). Another PDGFR-selective inhibitor, CP-673451, has demonstrated inhibitory effects on the proliferation and migration of lung cancer cells. Moreover, CP-673451 has exhibited the capacity to enhance the cytotoxicity of cisplatin and induce apoptosis in non-small cell lung cancer (20). In a phase II trial, Olaratumab®— (a human anti-PDGFR-α monoclonal antibody)-displayed an acceptable safety profile in patients with metastatic gastrointestinal stromal tumors (21). These instances underscore the therapeutic potential of targeting the PDGF pathway for the treatment of various malignancies. In this investigation, we focused on the design and construction of a single-chain PDGF receptor antagonist. This antagonist was strategically engineered such that one of its two poles retained the capability to bind with the receptor while the other pole lacked this ability. The intended outcome was to impede receptor dimerization, thereby inhibiting the PDGF signaling pathway. This inhibitory effect is achieved by displacing specific amino acid residues within PDGF BB that play a crucial role in the interaction between PDGF and its receptor. This targeted interference was informed by a meticulous analysis of the structure of the receptor-ligand complex, as illustrated in Scheme 1.
Scheme 1. Mechanism of Designed Single-Chain Antagonistic PDGF, which binds to one monomer PDGFR, prevents dimerization of receptors and therefore inhibits the PDGF signaling pathway.
MATERIALS AND METHODS
Materials
Isopropyl β-D-1-thiogalactopyranoside (IPTG) and kanamycin were procured from Invitrogen (Carlsbad, CA, USA). Nickel-nitrilotriacetic acid (Ni-NTA) affinity chromatography resin was supplied by Qiagen (Hilden, Germany). A 96-Well plate, specifically Max-iSorp, was provided by Nunc (USA). Oxidized glutathione was purchased from AppliChem (USA), while reduced glutathione was acquired from BioBasic (Canada). (3-(4,5-dimethyl thiazolyl-2)-2,5-diphenyltetrazolium bromide) MTT was obtained from Sigma (USA). Escherichia coli strain BL21 (DE3) was procured from Novagen (Madison, WI, USA) and New England Biolabs Inc. (Beverly, MA, USA), respectively. Cell culture medium was sourced from Bioidea Company (Tehran, Iran), and fetal bovine serum was acquired from Gibco/Invitrogen (Carlsbad, CA, USA). A549 cells were obtained from the American Type Culture Collection (ATCC; Manassas, VA, USA). All other chemicals used in the study were obtained from Merck (Darmstadt, Germany). YASARA software version 14.12.2 was employed for visualizing protein figures.
Design of PDGF Antagonist
The crystal structures of the PDGF-PDGF Receptor complex (PDB ID: 3MJG) were obtained from the Protein Data Bank (PDB), ensuring a reliable foundation for subsequent analyses. The CFinder server (http://bioinf.modares.ac.ir/software/nccfinder/) was used to identify the residues that are critical in the interaction between PDGF and PDGFR that cause receptor dimerization.
Residue Analysis and Replacement Strategy
For a detailed exploration of the residues engaged in protein-protein interactions within the PDGF-PDGF Receptor complex, the CFinder server was employed. This computational tool utilizes the protein complex PDB file as input, relying on accessible surface area differences (delta-ASA) to identify residues that contribute to ligand-receptor interactions. Subsequently, to facilitate the replacement of PDGF segments involved in binding to PDGFR, peptide segments with comparable geometry but distinct physicochemical properties were selected.
Protein Design and Fragment Replacement Strategy
The ProDA (Protein Design Assistant) server (http://bioinf.modares.ac.ir/software/proda) was instrumental in this process (22). This server aids in the identification of peptide segments suitable for substitution, ensuring the maintenance of structural integrity while introducing variations in physicochemical characteristics. This integrated approach, combining CFinder and ProDA servers, enhances our understanding of the intricate molecular interactions within the PDGF-PDGF Receptor complex and guides the design of the single-chain PDGF receptor antagonist with targeted modifications for disrupting receptor dimerization. The ProDA (Protein Design Assistant) web server, integral to our study, provides a comprehensive list of diverse protein segments by querying a database using specified input parameters. The criteria employed in the search encompass the number of amino acid residues, amino acid sequence patterns, secondary structure, distance between fragment ends, as well as the polarity and accessibility patterns of amino acid residues. The selection of suitable fragments is meticulously carried out based on several considerations, including amino acid content and specific characteristics such as secondary structure features, polarity, and accessibility patterns. These selected fragments from the candidate sequences are then strategically chosen for replacement within the PDGF BB sequence. This sophisticated approach, combining criteria-driven segment selection with subsequent integration into the PDGF BB sequence, ensures a thoughtful and targeted modification strategy in the design of our single-chain PDGF receptor antagonist.
Linker Design and 3D Structure Construction
To optimize purification and refolding processes while minimizing interference with the three-dimensional structure of the single-chain PDGF (sc-PDGF), an 18-amino acid residue linker was meticulously designed. This linker serves as a critical bridge between the two monomers of PDGF BB. Subsequently, the three-dimensional structure of the modified PDGF was constructed based on its primary sequence. The MODELLER software (version 9.17) (23) was employed for this purpose, generating a pool of 100 models. The model selection process involved choosing the model with the lowest MODELLER objective function score, indicating the best structural fit. To ensure the structural integrity and quality of the selected model, stereochemistry checks were performed using PROCHECK software (24). This rigorous validation step guarantees the reliability and accuracy of the constructed 3D structure, which is essential for subsequent analyses and experimental applications.
Molecular Dynamics Simulations
Molecular dynamics (MD) simulations were conducted employing GROMACS 5.0.7, focusing on both the modeled single-chain PDGF (sc-PDGF) and the native isoform. The simulations spanned a duration of 20 nanoseconds, utilizing the Gromos96 force field (25). The structure was solvated in a solvation box using a simple point-charge water model (26), with a minimum distance of 10 Å between the protein and the edges of the box. The system was neutralized by adding Cl– and Na+ ions that were randomly replaced with water molecules. The system was initially relaxed, and any bad contacts between atoms were removed through the steepest descent algorithm in an energy minimization step. The minimized systems were then equilibrated for 100 picoseconds (ps) using canonical and isothermal–obaric ensembles. The simulations were performed at 300 K and 1 bar. Finally, the equilibrated systems were simulated for a period of 20 nanoseconds (ns) with a 2-femtosecond (fs) time step to determine the possible effects of modification on the structure of sc-PDGF. The Root Mean Square Deviation (RMSD) and radius of gyration of the system were investigated and evaluated to determine the stability of the MD simulations and the compactness of the sc-PDGF during the simulations.
Molecular Docking Analysis
To assess the binding capabilities of both the native and modified PDGF with PDGFR, molecular docking simulations were conducted using the ClusPro server (https://cluspro.org) (27). Molecular Docking was performed with a monomeric receptor, and the ability of native/ modified PDGF to bind to the receptor was evaluated depending on the ClusPro score, and the results of Docking were evaluated.
Construction, Expression, Refolding, and Purification of Antagonistic PDGF
The PDGF antagonist-encoding gene was synthesized and subsequently cloned into the pET28a expression vector, flanked by BamHI/XhoI restriction sites. This molecular construct was facilitated by Shine Gene Molecular Biotech, Inc. (Shanghai, China). The steps involved in the construction, expression, refolding, and purification of the PDGF antagonist are detailed below:
Gene Cloning and Transformation
The synthesized PDGF antagonist-encoding gene was cloned into the pET28a expression vector, which was then transformed into Escherichia coli BL21 (DE3) cells.
Expression Conditions
The transformed cells were induced for expression at 37 °C, with 0.5 mM isopropyl β-D-1-thiogalactopyranoside (IPTG) for 6 hours.
Inclusion Body Collection and Dissolution
Inclusion bodies containing the expressed PDGF antagonist were collected and dissolved in 6 M urea.
Purification and Refolding
Purification and refolding were conducted using a previously described protocol (28). Column chromatography was employed with sequential elution using buffers A, B, C, D, and E respectively;
Buffers A: 6 mol/L urea, 0.5 mol/L NaCl, 10% glycerol, 1% TritonX-100, 20 mM Tris, pH 6.5.
Buffers B: 6 mol/L urea, 0.5 mol/L NaCl, 10% glycerol, 1% TritonX-100, 20 mM Tris, pH 5.8.
Buffers C: 4 mol/L urea, 0.5 mol/L NaCl, 6% glycerol, 20 mM Tris, 2 mM reduced glutathione (GSH), pH 8.0.
Buffers D: 2 mol/L urea, 0.5 mol/L NaCl, 3% glycerol, 20 mM Tris, 2 mM GSH, 0.2 mM oxidized glutathione (GSSG), pH 8.0.
Buffers E: 0.5 mol/L NaCl, 20 mM Tris, 2 mM GSH, 0.5 mM GSSG, pH 8.0. The elution buffer contained 300 mM imidazole and 0.5 mol/L NaCl.
Elution and Gel Analysis
The eluted modified PDGF was collected in sterile vials. The collected fractions were loaded onto an electrophoresis gel for further analysis. This detailed procedure outlines the steps taken to construct, express, and purify the modified PDGF antagonist, ensuring its structural integrity and functionality for subsequent experiments.
Circular Dichroism Measurement
CD spectra were performed using a spectropolarimeter (Jasco J-715, Japan) at the far-UV wavelength of 195-240 nm (sc-PDGF concentration was 0.1 mg/ml in phosphate saline buffer), to confirm that the secondary structures of refolded sc-PDGF were not significantly changed. The data were smoothed by the Jasco J-715 software to reduce the routine noise and calculate the secondary structure percentage of antagonistic PDGF. The results were reported as molar ellipticity [θ] (deg cm2.dmol-1), based on a mean amino acid residue weight (MRW) of sc-PDGF. The content of secondary structures of sc-PDGF was obtained and compared to those of modeled sc-PDGF and the crystal structure of native PDGF BB.
Growth Inhibition Assay
The inhibitory activity of modified PDGF was studied on adenocarcinomic human alveolar basal epithelial cells (A549). The cells were cultured in DMEM with 10% FBS and incubated in 5% CO2 at 37 °C. For growth inhibition assay, cells were collected by washing with PBS, added trypsin, then counted and 6000 cells/well were seeded in a sterile 96-well plate. After 24 h, the medium was replaced with fresh medium containing different concentrations of modified PDGF. The cells were incubated for 24 h at 37 °C. Afterward, cell growth inhibition was analyzed using the MTT assay. 10 µl of 5 mg/ml MTT solution was added to each well and the plates were incubated for 3-4 h at 37 °C. After that, the media were replaced with 100 µl of DMSO (dimethyl sulfoxide), and the absorbance of the wells was measured at 570 nm using a µQuant microplate reader (BioTek, USA) (29).
RESULTS
The design of the PDGF antagonist was informed by an analysis of accessible surface area (ASA) differences, identifying critical peptide segments and amino acid residues in PDGF BB involved in receptor binding. The fragments exhibiting the highest delta-ASA were recognized as crucial in the binding process to the receptor. Specifically:
1. In PDGF (subunit I): Fragments 13IAE15, 54NNRN57, and 98KCET101 were identified as essential for binding to the receptor (Figure 1A).
Based on these findings, strategic replacements were decided upon in four important regions (Figure 1C):
In the design of the single-chain PDGF (sc-PDGF), a linker sequence VGSTSGSGKSSEGKGEVV was incorporated. This linker serves to connect the C-terminus of subunit I of PDGF BB to the N-terminus of subunit II. The construction of the designed sc-PDGF was executed using MODELLER, and the best structure was meticulously selected for further analyses (Figure 1D). This refined sc-PDGF structure incorporates strategic modifications and a linker sequence to enhance its functional properties, setting the stage for subsequent evaluations. Figure 1. PDGF BB binding sites determined by C Finder. Critical amino acid residues in the binding receptor in subunit I (A) and II (B) of native PDGF, 3D structure of native PDGF BB (C), and 3D structure of single-chain PDGF (D). The candidate binding sites to be modified, the substituted amino acid residues, and the linker are shown in red, green and yellow, respectively.
Table 1. PDGF BB fragments are supposed to be modified, and fragments that replace them have similar geometry and secondary structure but different physicochemical properties.
The 3D structure of the designed single-chain PDGF (sc-PDGF) was modeled based on the crystal structure of wild-type PDGF BB. The structure with the lowest MODELLER objective function was selected for molecular dynamics (MD) simulations. The objectives of the MD simulations were to refine the sc-PDGF structures under similar conditions, compare them with native PDGF BB, and allow conformational relaxation before the docking study. After the simulations, the Root Mean Square Deviation (RMSD) and radius of gyration values for the backbone atoms of sc-PDGF were monitored relative to the starting structure during the MD production phase. The RMSD curves (Figure 2) indicated that the backbone atoms of the sc-PDGF structures were stable and reached equilibrium after 10 ns of simulation. Both structures exhibited RMSD values with no significant deviation. Additionally, the radius of gyration for the modeled sc-PDGF during the simulations showed negligible changes, indicating minimal alterations in the compactness of the proteins (Figure 2). These results affirm the stability and structural integrity of the modeled sc-PDGF during MD simulations, providing a solid foundation for subsequent analyses.
Figure 2. Molecular dynamic simulations result, RMSD and radius of gyration of the proteins during the simulations. RMSD (A) and radius of gyration (B) values of the backbone atoms of native PDGF BB (black) and sc-PDGF (gray) structures with respect to the reference coordinate during 20ns simulations.
The binding ability of the modified PDGF to the receptors was predicted using ClusPro and compared with native PDGF. The docking results revealed distinctive features between native PDGF and the modified PDGF: Native PDGF demonstrated two high-score positions capable of binding to PDGF receptors (PDGFRs). These positions were located on two symmetrical binding sites at its two poles. The modified PDGF exhibited only one high-score position, aligning with the anticipated outcome. As expected, the modified interface of sc-PDGF lost its ability to bind the receptor, and the modified PDGF could only bind to PDGFR through one pole with a high score. Consequently, the dimerization of receptors cannot take place (Figure 3).
Table 2. Protein-Protein Interaction Prediction by ClusProThese results from ClusPro, as summarized in Table 2, confirm the differential binding scores and binding sites between native PDGF and the modified sc-PDGF. In Cluster 0, both native PDGF and modified PDGF show high scores for binding to one pole, with the intended binding site for sc-PDGF. In Cluster 1, native PDGF exhibits a high score for the symmetrical pole, while the modified PDGF shows a low score, indicating altered binding characteristics. The antagonistic sc-PDGF does not display any binding on the modified pole, supporting its role in preventing receptor dimerization.
Figure 3. Protein-Protein Docking results ClusPro. The interaction between native PDGF BB and PDGFR. Native PDGF BB can bind with the receptor (yellow) by its own two equal poles shown in red (A), and the interaction between sc-PDGF and PDGFR, can only bind with the receptor by its unchanged pole (red sites). Substituted fragments cannot bind to the receptor, shown in green (B).
The synthesis and expression of the modified PDGF-encoded gene were carried out in E. coli BL21 (DE3). Subsequent steps in the construction of active antagonistic PDGF involved the collection and washing of insoluble inclusion bodies with plate wash buffer. The inclusion bodies were then dissolved using a solution buffer, filtered through a 0.22 μm filter, and loaded onto a Ni-NTA agarose column. Purification and refolding processes were performed concurrently on the column, and finally, 0.5 ml eluted samples were collected.
The success of the purification process was confirmed through SDS-PAGE analysis, as depicted in Figure 4.
Figure 4. SDS-PAGE analysis of the expressed and purified sc-PDGF. Inclusion body in protein expression obtained from E. coli BL21 (DE3) (A) and SDS-PAGE results of refolding and purification on Ni-NTA affinity chromatography column. Lanes 1-4, eluted fractions collected from Ni-NTA affinity column (B).
The secondary structure contents of the single-chain PDGF (sc-PDGF) were calculated using the CD spectrum and compared to the predicted model and the crystal native structure. The results, as presented in Table 3, indicate slight differences between the calculated secondary structure contents of sc-PDGF and the predicted model and crystal native structure.
Table 3. Secondary structure contents of sc-PDGF obtained by CD compared to predicted from modeled sc-PDGF and crystal PDGF BB 3D structure.Anti-proliferation effect of Antagonistic PDGF
A cell viability test was conducted using A549 cells to assess the inhibitory effect of modified PDGF. The experiment involved incubating and treating 6000 A549 cells with different concentrations of the modified PDGF (Figure 5). The results indicate a dose-dependent inhibitory effect on cell proliferation; At a concentration of 0.25 μg/ml of PDGF antagonist, there was approximately a 30% inhibition of A549 cell proliferation compared to the control; a concentration of 0.75 μg/ml of PDGF antagonist resulted in approximately a 50% inhibition of cell growth; the highest concentration tested, 3 μg/ml of PDGF antagonist, resulted in a remarkable inhibition of cell proliferation, reaching up to about 90%. The concentration that inhibits 50% of cell proliferation (IC50) was calculated using Prism software and found to be 0.7151 μg/ml (27.7 nM).
Figure 5. Anti-proliferation Effect of Antagonistic PDGF on A549 Cells. Each concentration was performed with 3 replicates, error bar ≈ ± SD (standard deviation).
These results demonstrate the potent anti-proliferative activity of the modified PDGF antagonist on A549 lung cancer cells, indicating its potential as a therapeutic agent for inhibiting cancer cell growth.
DISCUSSION
The inhibition of the platelet-derived growth factor (PDGF) signaling pathway has been identified as a crucial target for the treatment of various malignant and nonmalignant diseases, including cancer and fibrotic diseases, where PDGF plays a pivotal role. The selective inhibition of the PDGF signaling pathway offers numerous advantages in the treatment of diverse diseases, minimizing potential side effects on other cells (16). In this study, we focused on designing and constructing a single-chain PDGF receptor antagonist, aiming to disrupt the dimerization of PDGF receptors and subsequently inhibit the PDGF signaling pathway. This approach is significant given the central role of PDGF in physiological and pathological conditions. The designed single-chain antagonistic PDGF (sc-PDGF) was constructed based on structural information derived from the PDGF BB/receptor complex. Molecular dynamics simulations and structural analyses were employed to evaluate the binding affinity and stability of the sc-PDGF mutant interface. The successful expression, purification, and refolding of sc-PDGF were confirmed through various techniques, including far-UV CD spectroscopy. The molecular docking results showed that sc-PDGF had a reduced ability to bind to PDGF receptors compared to native PDGF, supporting its potential as an effective antagonist. The calculated secondary structure contents of sc-PDGF, obtained through CD spectroscopy, indicated minimal changes, further affirming the structural integrity of the designed antagonist. Furthermore, the anti-proliferation assay demonstrated the potent inhibitory effect of sc-PDGF on A549 lung cancer cells in a dose-dependent manner. The calculated IC50 value highlighted the concentration at which 50% of cell proliferation was inhibited. This study provides valuable insights into the development of a targeted therapeutic approach for diseases associated with aberrant PDGF signaling. The designed sc-PDGF shows promise as a selective antagonist with potential applications in the treatment of cancer and fibrotic diseases, offering a novel avenue for the development of targeted therapies with minimized off-target effects. Future investigations may focus on in vivo studies and clinical applications to validate the therapeutic efficacy of the designed sc-PDGF. Current PDGF antagonists, particularly small molecule kinase inhibitors such as Imatinib, exhibit non-selectivity, leading to undesired side effects on various tissues (3, 29). Additionally, antibodies, while effective, come with high costs and may stimulate the immune system, posing potential challenges (29, 30). In our research, we aimed to develop a selective PDGF antagonist. The PDGF signaling pathway is initiated by the dimerization of PDGF receptors through dimeric PDGF. In our study, we focused on modifying one pole of the PDGF dimer, allowing the antagonistic PDGF to bind exclusively to one receptor. This modification prevents receptor dimerization, subsequently selectively inhibiting the PDGF signaling pathway. In a similar approach, Ghavami et al. successfully designed and synthesized a potent VEGF antagonist capable of inhibiting angiogenesis and preventing capillary tube formation in HUVEC cell lines (31). This strategy of selectively targeting specific pathways by modifying critical interaction sites has shown promise in controlling pathological processes. Our engineered sc-PDGF antagonist, designed to disrupt the dimerization of PDGF receptors, holds the potential for selective inhibition of the PDGF signaling pathway. This approach provides a novel alternative to existing PDGF antagonists, addressing issues related to non-selectivity and cost associated with current therapeutic options. Further studies, including in vivo investigations and clinical trials, will be crucial to validate the therapeutic efficacy and safety profile of the designed sc-PDGF. We identified the crucial amino acid residues responsible for binding to the receptor at one pole of PDGF BB within the shared interface of two subunits (Figure 1). Subsequently, we modified these residues to hinder binding, specifically -replacing Glu15 with Lys, introducing an opposite charge. We replaced the segment 54NNRN57 with ADED, which has opposite physicochemical properties while maintaining the same geometry. Additionally, the two crucial binding fragments, 25-43 and 74-85, in the other subunit were replaced with two turns. These turns were carefully selected from a database to ensure that they maintained the original geometry without amino acid residues that bind to the receptor (Table 1). The PDGF BB isoform was chosen due to its ability to bind and activate all PDGF receptor types (αα, ββ, and the heterodimer complex αβ). Furthermore, the crystal structure of the PDGF BB/PDGFR complex has been elucidated. We determined the sequence of the engineered sc-PDGF antagonist and modeled its 3D structure (Figure 1D). Molecular dynamics simulations were conducted on the modeled sc-PDGF to facilitate the conformational relaxation of its structure before the docking study. The RMSD and radius of gyration values indicated stable behavior with no significant deviation, as illustrated in Figure 2. Furthermore, the docking binding scores of both native and modified sc-PDGF to the receptor indicate a noteworthy difference. The native PDGF exhibits two high-scoring positions precisely on the expected sites, whereas the sc-PDGF shows only one high-scoring position (Table 2 and Figure 3). This suggests that the modified interface may have lost its ability to effectively bind to the receptor. The coding sequences of the sc-PDGF gene were synthesized and incorporated into pET28a expression vectors. Subsequently, E. coli BL21 (DE3) was transformed, and the modified sc-PDGF was expressed and refolded as outlined in the methods section. The presence of a linker between two PDGF monomers and a His tag at the N-terminus facilitated the purification and refolding process, streamlined by the Ni-NTA affinity chromatography column, as illustrated in Figure 4. The designed PDGF antagonist exhibited inhibitory effects on A549 cell proliferation, with a concentration of 3 µg/ml causing a notable reduction in cell growth to 10% compared to the control (Figure 5). This observation underscores the antagonist’s inhibitory impact on PDGFR, achieved through the prevention of receptor dimerization. Furthermore, the modified pole of sc-PDGF lost its ability to bind to the receptor, confirming the intended impact. According to the ClusPro docking results, sc-PDGF is predicted to have lost the ability to bind to two receptor molecules simultaneously. This loss is crucial in the context of PDGF dimerization and signaling, aligning with the findings from MTT assays. The results confirm the inhibitory effect of the antagonistic sc-PDGF on A549 cell lines, which is consistent with previous research. In a related study, demonstrated that inhibiting the PDGF receptor can effectively suppress cell growth in the A549 cell line (32). Our study’s notable advantage lies in the extracellular mechanism of inhibition, which has the potential to prevent cellular uptake. This approach addresses the challenges associated with cellular uptake, as well as intracellular metabolism and degradation of the drug (33, 34). Furthermore, the high selectivity of the designed antagonistic PDGF suggests a potential reduction in side effects on other cells. In a related study, Boesen et al. [reference] prepared single-chain variants of VEGF by incorporating a 14-residue linker between two monomers. Their findings demonstrated that these single-chain variants were fully functional and equivalent to the wild-type VEGF. In their work, Zhao et al. also successfully prepared an effective single-chain antagonist of VEGF (35). This was achieved by deleting and substituting critical binding site residues in one monomer of the native VEGF while keeping the other monomer intact. This strategic modification prevented the dimerization of the receptors, consequently inhibiting the VEGF signaling pathway (35). In parallel studies, Khafaga et al. and Qin et al. designed antagonistic VEGF variants by structurally analyzing VEGF and modifying amino acid residues at the binding site on one pole of the protein (36, 37). They successfully produced antagonistic single-chain VEGF and confirmed its inhibitory effect. Additionally, Kassem et al. demonstrated the antagonization of growth hormone (GH) by preventing receptor dimerization (38). This was achieved through the binding of one receptor molecule by monovalent fragments of GH, effectively preventing receptor dimerization and inhibiting the signaling pathway (39). Activation of PDGF receptors, similar to VEGF and growth hormone receptors, necessitates binding of ligands at two distinct sites to initiate receptor dimerization. Consequently, by deleting or modifying one binding site while preserving the other, the ligand occupies only one receptor, preventing the dimerization of receptors. This strategic modification inhibits the cascade phosphorylation of the receptor and its subsequent effects. In conclusion, PDGF signaling inhibitors have demonstrated efficacy in various clinical applications, particularly in certain cancers and fibrotic diseases. The engineered sc-PDGF antagonist, designed to bind to a single receptor, effectively prevents the dimerization of PDGFRs and inhibits their signaling pathway. Docking results highlighted the inability of the modified PDGF to bind on one pole while retaining binding on the other. The proliferation assay confirmed the inhibitory effects on A549 cells, suggesting that the sc-PDGF antagonist could serve as a potential therapeutic agent for diseases involving the PDGF signaling pathway.
INTRODUCTION
Angiogenesis, the branching out of new blood vessels from pre-existing vasculature, occurs physiologically during embryogenesis, the female reproductive cycle, and wound healing (1). It is also a crucial process in a variety of pathological conditions, including tumor growth, metastasis (2, 3), ischemic diseases, diabetic retinopathy (4, 5), chronic inflammatory reactions, age-related macular degeneration, rheumatoid arthritis, and psoriasis (6). Vascular endothelial growth factor (VEGF) is the most potent and predominant regulator of angiogenesis described to date (7, 8). This angiogenesis factor can instigate numerous biological responses in endothelial cells (ECs), such as survival, proliferation, migration, and vascular permeability, as well as the production of proteases and their receptors, creating prime conditions for angiogenesis (9, 10). It is estimated that up to 60% of human cancer cells express VEGF to create the vascular network necessary to support tumor growth and metastasis. Inhibiting angiogenesis has become an intensely investigated pharmaceutical area and represents a promising strategy for the treatment of cancer and several other diseases (11). Because of its central role in pathological angiogenesis, VEGF is a major therapeutic target. Strategies aiming to block the binding of VEGF to its receptors or to block intracellular signaling events form the basis of many new developments in anti-angiogenic cancer therapy (12). Numerous substances have been developed as angiogenesis inhibitors, some of which have already been approved for clinical use. These include monoclonal anti-VEGF antibodies (bevacizumab and ranibizumab) (13), anti-VEGF aptamers (pegaptanib), and VEGF receptor (VEGFR) tyrosine kinase inhibitors (sorafenib and sunitinib) (14, 15). Single-domain antibodies, also known as nanobodies or VHHs, possess valuable characteristics such as effective tissue penetration, high stability, ease of humanization, efficient expression in prokaryotic hosts, and high specificity and affinity for their respective antigens. Consequently, they can be introduced as alternative therapeutic candidates to traditional antibodies. VHHs represent the smallest functional unit of an antibody, preserving all of its functions, and due to their minimal size, they are also recognized as nanobodies (16, 17). Studies conducted by Shahngahian and colleagues in 2015 demonstrated that VEvhh10 (accession code LC010469) has a potent inhibitory effect on the binding of VEGF to its receptor (18). This VHH exerts its inhibitory role by binding to the VEGF receptor binding site. Among the members of the VHH phage display library, VEvhh10 possesses the highest binding energy at the VEGF receptor binding site, covering vital amino acids involved in the biological activity of VEGF and disrupting its function (18). The conventional expression of nanobodies in E.coli faces several challenges, primarily stemming from their small size and complex folding requirements. Nanobodies often exhibit low solubility, leading to the formation of inclusion bodies and hampering their functional utility. Moreover, the intricate disulfide bond formation and protein folding pathways of nanobodies make them prone to misfolding and aggregation within the bacterial cytoplasm (19-21). One standard method for expressing non-fused VHH is the use of E. coli expression systems. However, expressing non-fused VHH using conventional cytoplasmic expression methods in this prokaryotic system often faces challenges, including low expression, poor solubility, and misfolding of the antibody in E. coli (22-24). To overcome these shortcomings, we chose a novel expression system using a small ubiquitin-related modifier (SUMO) molecular partner (25, 26). SUMO Fusion Technology has emerged as a powerful tool for enhancing the soluble expression of proteins, including nanobodies, in E. coli. By fusing the target protein with the SUMO protein, researchers can promote proper folding, enhance solubility, and increase expression yields. SUMO fusion tags facilitate protein purification and can be cleaved post-purification to yield the desired protein product in its native form (27). SUMO is covalently attached to other proteins and plays roles in post-translational modifications (28). These roles include significantly increasing the yield of recombinant proteins, facilitating the correct folding of the target protein, and promoting protein solubility (29). The aim of this study was to develop an alternative method for more efficient production of VHH nanobodies in an E. coli-based expression system using the SUMO fusion tag. The SUMO fusion tag improves the solubility and yield of VHHs. Our results demonstrated that SUMO is very effective in promoting the soluble expression of VHH in E. coli. The resulting recombinant bioactive VHH can be used for therapeutic applications and clinical diagnosis in the future.
MATERIALS AND METHODS
Molecular and Chemical Materials
The materials, chemicals, and reagents required for the lab are listed as follows:
Ampicillin, kanamycin, and agarose from Acros (Taiwan), IPTG from SinaClon (Iran)
Ni-NTA resin from Qiagen (Netherlands), Plasmid extraction kit and gel extraction kit from GeneAll (South Korea), Enzyme purification kit from Yektatajhiz (Iran), Restriction enzymes HindIII/XhoI, ligase enzyme, and other molecular enzymes from Fermentas (USA), Pfu polymerase and Taq polymerase from Vivantis (South Korea), Primers from Sinagen (Iran), Methylthiazole-tetrazolium (MTT) powder from Sigma (USA), Penicillin-Streptomycin, Trypsin-EDTA, and DMEM-low glucose from Bio-Idea (Iran), Fetal Bovine Serum (FBS) from GibcoBRL (USA), Monoclonal conjugated anti-human antibody with HRP from Pishgaman Teb (Iran), Anti-Austen antibody from Roche (Switzerland), Other chemicals from Merck (Germany). Schematic 1 shows a schematic diagram of the construction of the pET-28a-SUMO-TEV-VEvhh10 Gene using SnapGene v5.1.5.
First, suitable primers were designed by OligoAnalyzer to isolate the SUMO gene sequence (see Table 1). The plasmid was used as a template for the polymerase chain reaction (PCR). Amplification was performed using the Pfu polymerase enzyme in a thermal cycler, utilizing the software we designed for this research (see Table 2). Different temperatures were tested (58, 59.5, 61, 62.5, 64) for primer annealing to the PCR template, with 58 °C being selected as the optimum temperature. The restriction enzyme sites were incorporated at the beginning and end of the primers. Additionally, the TEV protease cleavage site was placed between the SUMO and VEvhh10 sequences.
Gene Cloning: Primers for the amplification of the VEvhh10 gene with the accession code LC010469 were initially designed (Table 3). The plasmid containing the gene fragment served as a template for the PCR reaction. Amplification was carried out using Taq polymerase and Pfu polymerase enzymes in a thermocycler with a programmed temperature profile (Table 4). Various annealing temperatures for primer binding to the template were tested, and a temperature of 65°C was found to be the optimal annealing temperature. Primer cutting sites at the beginning and end of the Gene were designed, and the location of the TEV protease enzyme cutting site was positioned between the SUMO and the VEvhh10 sequence.
The gene fragments of SUMO and VEvhh10, amplified using Pfu polymerase via PCR, were extracted from the gel. Simultaneously, the amplified fragments and the pET-28a vector were digested using the BamHI and XhoI restriction enzymes. Purification was carried out using a commercial enzyme purification by GeneAll kit. Subsequently, the gene fragments were ligated into the target vector using T4 DNA ligase enzyme. The resulting ligated product was incubated overnight at 4°C. The ligated product was then transformed into E. coli DH-5α bacteria. To confirm the insertion of the Gene into the vector, the transformed bacteria were plated on kanamycin antibiotic-containing LB agar plates. Finally, the obtained colonies underwent Colony PCR using specific primers targeting VHH, T7 promoter, and terminator regions. The PCR products were analyzed on a 1% agarose gel, and positive transformants were screened and cultured further. The non-recombinant plasmid was purified using a plasmid extraction kit, and enzymatic digestion was performed to confirm the insertion of the gene fragment into the plasmid.
Expression and Soluble Detection of Recombinant SUMO-VHH
As depicted in Schematic 2, the pET-28a plasmid containing SUMO and VHH was transformed into E.coli BL21 (DE3) expression host cells using the heat shock method. The accuracy of transduction was confirmed by isolating colonies grown on LB agar medium supplemented with 50 mg/mL kanamycin. To express the genotype protein, a colony of bacteria containing the recombinant expression plasmid was inoculated into 10 mL of LB culture medium supplemented with 50 mg/mL kanamycin antibiotic and incubated at 37°C with optimal aeration at 250 rpm. Subsequently, 1 mL of mature bacteria was transferred to 50 mL of LB medium containing kanamycin antibiotics and incubated at 37°C until the OD600 reached approximately 0.6. Expression was induced by adding 0.5 mM IPTG and continued for 22 hours at 25°C. Following expression, the resulting product was centrifuged at 4000 rpm for 15 minutes at 4°C, and the bacterial cells were sonicated in lysis buffer (pH 8). The sonication product was centrifuged again at 12,000 rpm for 20 minutes at 4°C, and the supernatant was analyzed using the SDS-PAGE method (30). Proteins were purified by gradient chromatography using a nickel agarose column. The protein sample was transferred to the column pre-equilibrated with wash buffer (50 mM Tris-base, pH 8.0, 300 mM NaCl, 20 mM imidazole). Proteins that did not bind to the column due to the lack of histidine sequence were removed. Only the target protein remained attached to the column due to the presence of the histidine sequence. The bound proteins were eluted using elution buffer (50 mM Tris-base, pH 8.0, 300 mM NaCl, 250 mM imidazole). Purification was conducted using cold buffers to prevent thermal degradation of the proteins. Protein concentration was determined using the Bradford method with BSA as the protein standard (31).
The expression and purification of VEGF8-109
The His-tagged VEGF8-109-RBD was expressed in E.coli BL21 (DE3) bacterial cells and subsequently purified using a Ni-NTA agarose column, following previously described protocols with slight modifications (32). Protein expression and purification were analyzed through sodium dodecyl sulfate-polyacrylamide gel electrophoresis (SDS-PAGE) and Coomassie Brilliant Blue R250 staining. To remove excess salt, the purified protein was dialyzed three times against phosphate-buffered saline (PBS) containing 10% (v/v) glycerol at 4 °C for 12 h. Protein concentration was estimated using the Bradford assay, with BSA as a standard (31).
ELISA-Based Immunoassay
The designed immunoassay is shown in Schematic 3. First, 100 μL of SUMO-VHH recombinant protein in carbonate-bicarbonate buffer was added to each well of a cell ELISA and incubated for 16 hours at room temperature. After 16 hours, the supernatant solution was dried and washed three times with 100 μL of PBS buffer. The blocking step was performed using a PBS solution containing 2% gelatin in a volume of 350 μL and placed at 37°C for 1 hour. Subsequently, the blocking buffer was dried and washed three times with 100 μL of PBST buffer (PBS + 0.05% Tween-20). Next, 100 μL of serially diluted VEGF solutions (ranging from 0.5 ng/mL to 1000 ng/mL) were added to the wells and incubated for 2 hours at room temperature. After incubation, all wells were dried and thoroughly washed three times with PBST buffer. Following this, 100 μL of human anti-VEGF monoclonal antibody at a concentration of 1000 ng/mL was added to the wells. After washing with PBST, 100 μL of HRP-conjugated anti-human IgG antibody was added to each well and incubated in the dark at 37°C for 1.5 hours. Subsequently, 100 μL of TMB was added to each well and incubated for 15 minutes in the dark. Finally, 100 μL of 2 N sulfuric acid was added to each well, and the absorbance of the wells was read at a wavelength of 450 nm (33).
RESULTS
Products of the PCR Reaction
Based on our previous study (34), the VEvhh10 gene was amplified using pfu polymerase at an annealing temperature of 65 °C as showed in (Fig. 1).
Construction of pET28a-SUMO-VHH Expression Plasmid
As depicted in (Fig. 3), the VEvhh10 product (402 bp) was amplified and double digested using HindIII and XhoI enzymes. The resulting product underwent purification in the preceding steps. The SUMO product, generated by the Pfu enzyme, was double digested using HindIII and BamHI enzymes, and the product (304 bp) was purified. Additionally, the plasmid pET28a was double digested using BamHI and XhoI enzymes, and the resulting product was also purified in preparation for the conjugation process.
After joining the aforementioned sequences with the presence of the T4 DNA ligase enzyme, the respective products were incubated at 4°C for 14 hours and transformed into E. coli DH5α. The transformed product was cultured on a kanamycin plate, as shown in (Fig. 4.b). Agar plates containing the antibiotic ampicillin were labeled as a negative control, as depicted in (Fig. 4.a). Subsequently, in (Fig 4. c), some samples were isolated and confirmed using colony PCR by specific primers related to the T7 Promoter and Terminator, as shown in (Fig 4.d), and specific primers related to VHH, as depicted in (Fig. 4.e).
Confirmation of Gene Cloning
A fresh single colony was grown in LB agar containing the antibiotic kanamycin, and the extracted plasmid was used as a template to amplify the target gene and construct the SUMO-VHH. This step aimed to confirm the correctness of the gene cloning stage. PCR was carried out using the Forward and Reverse primers of the VEvhh10 gene (414 bp) and SUMO gene (approximately 310 bp), as depicted in (Fig. 5.a), as well as the Forward T7 promoter Reverse SUMO primers (approximately 470 bp), as shown in (Fig. 5.b). To ensure further reassurance, the plasmid was double digested using the cutting enzymes BamHI and XhoI. The double enzymatic digestion process was performed, and the desired Gene was observed on the gel, as illustrated in (Fig. 5.c). The results indicated the success and confirmation of gene cloning.
Expression and Purification of the SUMO_VHH
E.coli cells harboring SUMO-VHH were induced by 0.5 mM IPTG for 22 h at 25°C. The cell pellets were harvested by centrifugation, and protein was extracted and separated by sonication and centrifugation. The supernatants and precipitate were collected and subjected to 12% SDS-PAGE analysis. As depicted in (Fig. 6), the expression of a 24 kDa protein, similar to the predicted size, was induced by IPTG, compared with the negative control (blank plasmid) or recombinant bacteria without IPTG induction.
Previous studies have indicated that recombinant antibodies often form inclusion bodies using traditional E. coli expression methods (35). Our results suggest that SUMO is very helpful in promoting the soluble expression of VHH (36). To obtain high-purity recombinant protein, Ni-NTA chromatography was employed for purification because the 6His-tag was located in the N-terminal of SUMO. Different concentrations of imidazole were tested to elute the recombinant protein. The results showed that SUMO-VHH was efficiently eluted from the Ni-NTA column using an elution buffer containing 250 mM imidazole. SDS-PAGE analysis demonstrated that the purity of SUMO-VHH exceeded 90%, as shown in (Fig. 7).
Expression and Purification of Recombinant VEGF 8-109
As indicated by our previous research, recombinant VEGFRBD8-109 was produced in soluble form in E. coli BL21 (DE3) strain after induction for 22 hours at 24°C. Purification of the His-tagged fusion protein was performed using Ni-NTA affinity chromatography. The activity of the unconjugated VEGF RBD 8-109 produced was also evaluated through its effect on the growth and proliferation of human umbilical vein endothelial cells (HUVECs) using the MTT assay (37, 38). Cell proliferation was good with an increase in the concentration of unfused VEGF RBD 8-109. At a concentration of 240 ng/ml, the cell count reached approximately 80% compared to the sample lacking VEGF8-109 (34). Accordingly, it can be used in VHH-SUMO binding assays.
Investigating the Binding Ability of Recombinant SUMO-VEvhh10 with VEGF RBD 8-109
To verify the binding ability of recombinant SUMO-VEvhh10 to VEGF, an ELISA-based immunoassay was utilized according to the method described earlier. The results of the dose-response curve of VEvhh10-SUMO expressed using the ELISA method (Fig. 8) demonstrated that as the VEGF concentration increased, more anti-VEGF molecules were attached, resulting in increased light absorption.
DISCUSSION
The success of this study in amplifying, cloning, and expressing the VEvhh10 and SUMO genes underscores the utility of pfu polymerase and enzyme-based digestion for accurate gene construction as depicted in (Fig. 1), (Fig. 2), (Fig. 3) and (Fig. 5). The transformation of the ligated products into E. coli DH5α and subsequent colony PCR verification demonstrate that this method is efficient for cloning single-domain antibodies like VHH. The ability to amplify and clone the VEvhh10 gene with precision using pfu polymerase is consistent with earlier reports, which highlight the enzyme’s high fidelity in DNA replication and amplification compared to Taq polymerase. This methodological choice was critical in ensuring the accuracy of the VEvhh10 sequence during amplification (34). Moreover, the fusion of SUMO to VHH played a crucial role in enhancing the soluble expression of VHH. Previous studies have shown that recombinant VHHs often suffer from poor solubility and tend to aggregate into inclusion bodies when expressed in E. coli (35). The presence of SUMO, a small ubiquitin-like modifier, aids in overcoming this limitation by acting as a solubility-enhancing partner. SUMO fusions not only improve the solubility of the target nanobodies but also stabilize it during expression in bacterial hosts (36). In this study, SUMO fusion resulted in high yields of soluble VHH, which was efficiently expressed and purified using Ni-NTA chromatography with purity exceeding 90% as depicted in (Fig. 6) and (Fig. 7). This high purity, confirmed by SDS-PAGE, highlights the effectiveness of SUMO in both solubility enhancement and streamlined purification using His-tag. The successful production of recombinant VEGF 8-109 and its biological activity in promoting HUVEC proliferation further supports its use in functional assays. The MTT assay results, showing increased cell proliferation in response to VEGF 8-109, are consistent with previous research that indicates the importance of VEGF in promoting angiogenesis and endothelial cell growth (34). This validates the functional integrity of the expressed VEGF 8-109, suggesting that it retains its biological activity post-purification. The recombinant VEGF 8-109 can now serve as a valuable reagent in future binding assays with VHH or other therapeutic antibodies targeting VEGF pathways. Furthermore, the ELISA-based binding assays confirmed that SUMO-VEvhh10 binds effectively to VEGF, with increasing concentrations of VEGF resulting in a corresponding increase in light absorption as depicted in (Fig. 8). This binding affinity is crucial, especially for applications where VHH antibodies might be used to inhibit VEGF-mediated signaling, which is implicated in pathological conditions such as cancer and age-related macular degeneration (39). The observed binding behavior is consistent with previous works where VHH antibodies, despite their small size, exhibit strong binding capabilities to their respective antigens. This reinforces the potential of SUMO-VEvhh10 as a therapeutic agent targeting VEGF-related diseases, and the use of the SUMO fusion strategy could offer advantages over traditional expression systems by improving stability, solubility, and yield. Comparatively, the SUMO fusion system has demonstrated its superiority over other solubility-enhancing tags such as GST or MBP in maintaining the bioactivity of recombinant proteins (40, 41). While GST and MBP are effective in promoting solubility, they can sometimes mask epitopes or interfere with downstream applications, making SUMO a preferable option in cases where antigen binding and bioactivity must be preserved (42). This study adds to the growing body of literature that underscores the effectiveness of SUMO fusion in producing functional and soluble VHH antibodies for therapeutic applications. Future work could focus on optimizing expression conditions to further enhance yield or test the efficacy of SUMO-VEvhh10 in animal models of VEGF-related diseases.
CONCLUSION
This study highlights the utility of SUMO Fusion Technology for enhancing the expression of VEvhh10 targeting VEGF in E. coli. The successful production of soluble VHH against VEGF underscores its potential for future therapeutic and diagnostic applications in various diseases.
Journal:Syrian Journal for Science and Innovation
Abbreviation: SJSI
Publisher: Higher Commission for Scientific Research
Address of Publisher: Syria – Damascus – Seven Square
ISSN – Online: 2959-8591
Publishing Frequency: Quartal
Launched Year: 2023
This journal is licensed under a: Creative Commons Attribution 4.0 International License.