A Study of the Properties of Compost Garbage and its Effect on Germination and Breeding of Okra (Hibiscus Esculentus sp)
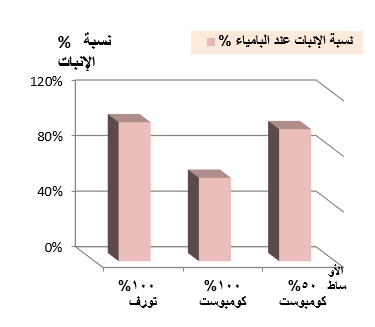
Introduction
The Internet of Things (IoT) makes all smart devices connect to the Internet anywhere at any time to form the Internet of Things [1]. Smart devices are described to be low power and limited capability of processor and memory. These devices can be linked together to form a network without an infrastructure in which nodes act as a router. Smart devices use IEEE 802.15.4 [2] and routing protocol RPL [3], but RPL did not support mobile nodes, so many researchers worked to improve it, but their results showed more overhead because of sending more control messages in the networks. other researches results were not accurate because of depending on RSSI value (Received signal strength indication) to detect the mobility of nodes, which is affected by obstacles and interference. Our contribution is to improve RPL protocol by making it able to ensure the nodes aware of the mobile node movement, so the node can reconnect to the network as soon as possible and reduce the disconnection time without increasing the transmission rate of control messages. The proposed protocol is lightweight and suitable for devices with limited and restricted specifications because it uses new control message which is not periodically sent.
The rest of this paper is organized as following: The first section introduces the RPL routing protocols, then related works are mentioned with discussions about the challenges of the research to design an efficient LLNs routing protocol. After that the proposed control message is explained, followed by the extensive simulation, performance evaluation and interpretations. Finally, the paper is concluded.
RPL (Routing Protocol for Low Power and Lossy Networks(LLN)) [3]:
In the network layer of the IoT protocol stack using 6LoWPAN technology, The RPL routing protocol was developed by (ROLL) group and was described in RFC (6550). RPL is suitable for fixed devices not the mobile devices. The root node serves as a gateway to the Internet for devices in the network. RPL organizes a topology as a Directed Acyclic Graph (DAG) that is partitioned into one or more Destination Oriented DAGs (DODAGs), one DODAG per root. It sends periodic DIO (DODAG Information Object) messages to inform nodes of its existence. It invites the neighbour nodes to call it, and in turn, each node that hears the DIO message and wants to communicate with root node will send a DAO (Destination Advertisement Object) message, then each node that communicates with parent node sends DIO messages to make the rest of the nodes connect and this process is repeated until all nodes are connected together, which is shown in Figure 1.
Fig. 1: Control messages used in RPL
The RPL protocol depends on the Trickle algorithm to manage the timers used to send periodic messages, to reduce the overhead on these networks. When the state of instability is detected, the transmission rate of control messages increases to spread updates quickly and reduces when the network is stable [4]. The node selects the parent node using Objective Function (OF) that depends on the number of hops, then it was developed to MARHOF (The Minimum Rank with Hysteresis Objective Function) [5] in which the parent node is selected based on the value of the Expected Transmission Count (ETX) that determine the quality of the link. The RPL protocol did not specify any mechanism for detecting routing adjacency failures of mobile node because such a mechanism causes overhead in bandwidth and power consumption. It is not suitable for Battery-powered devices to send periodic messages, so it requires external mechanisms to detect that the neighbour node is no longer reachable. This mechanism should preferably focus on links that were already used . [3]
Related work
Fotouhi (2015) proposed the MRPL protocol by integrating the RPL protocol with the smart hop using beacons. The protocol has two phases: the discovery phase and the data transmission phase. In the route discovery phase, the mobile node sends a broadcast of n DIS messages. The node that receives DIS messages calculates the ARSSI and adds this value within a DIO message. The mobile node selects its parent node that sent the DIO message with the highest ARSSI .[6] Gara (2016) suggested to use adaptive timer algorithm to regulate the transmission of DIO and DIS messages by the mobile nodes. The proposed algorithm computes (d) the remaining distance for a node to leave the parent node’s radio range by subtracting the preferred parent node’s radio range from the value of the distance between the two nodes. When (d) distance becomes shorter, the node discovers to find a new parent node. The researcher suggested using ETX and RSSI values to determine the best parent node .[7]Cobârzan (2016) proposed Mobility-Triggered RPL (MT-RPL) is a cross-layer protocol operating between the MAC and routing layers. It enables X-Machiavel operations to use information from layer 2 to trigger actions at layer 3 to ensure the node connects to the network. It reduces the disconnection time, increases the packet delivery ratio, and reduces overhead. A restriction of MT-RPL is that it relies on a fixed node that acts as an opportunistic forwarder for packets sent by a mobile node .[8]Wang (2017) proposed an RRD (RSSI, Rank, and Dynamic) method to develop the RPL protocol based on a combination of Received Signal Strength Indicator (RSSI) monitoring, Rank updating, and dynamic control message. It proposes a new DIO interval by modifying it dynamically according to Rank updates. RRD increased the packet delivery ratio and decreased the end-to-end delay and overhead, but it did not consider energy consumption .[9] Fotouhi (2017) proposed mRPL+ [10], when the link quality decreases during the transfer process with the parent node, the node will start sending periodic DIO messages to search for a better parent node. It also relied on the principle of overhearing to allow the parent node’s neighbour nodes listen for all messages exchanged in their radio range. When a neighbour node detects good link quality with the mobile node, it sends a DIO message to link with it. mRPL+ achieved good results in terms of the packet delivery rate, reaching 100%, but it led to more power consumption. Bouaziz and Rachedi (2019) [11] focused on two phases in the protocol, the first one is motion detection for the nodes, and the second is predicting the new connection before the current connection is lost. It relied on the principle of excluding the mobile nodes from the path to avoid interruptions caused by them and considered the mobile nodes as a leaf in the network that isn’t a router. EMA-RPL assumed that the node is moving away when the value of ARSSI decreases, but this is not always true because this value is affected by obstacles. Bouaziz (2019) [12] used the Kalman filter algorithm to predict the movement of mobile nodes and choose the parent node based on the predicted path. EKF-MRPL assumes that the mobile nodes will not participate in any routing process, but this is not always possible, especially in applications with many mobile nodes. Predicting movement and calculating distances are based on RSSI value which is inaccurate because it is affected by obstacles. Sanshi (2019) [13] modified the RPL protocol using fuzzy logic with several parameters (residual power, expected transfer count ETX, RSSI, mobility timer). FL-RPL uses the mobility timer parameter, which is the expected time to remain within the radio range depending on the location obtained from the RSSI value. This method isn’t accurate because it is affected by barriers and interference. Mobile nodes are considered leaf nodes and cannot participate in the routing process, This concept is not correct in the case of a network with more mobile nodes than fixed ones. Manikannan (2020) [14] used the firefly algorithm (FA) inspired by the firefly that produces light to communicate or attract prey or as a protective warning mechanism. When the distance increases, the light becomes weaker. It depends on choosing the parent node with a high RSSI value and re-implementing the FA algorithm until it reaches an optimal solution. In the simulation, only 12 nodes were used, and FA-RPL improved the packet delivery rate by 2.31%. The firefly algorithm (FA) is a good and powerful optimization algorithm, but its computational complexity is high .Safaei (2022) [15]. The research proposed ARMOR protocol by using a new parameter TTR to select the best parent node that will stay the longest within the radio range. TTR is calculated based on the node’s speed and position, and it is added to the DIO message. A new timer was added to increase the rate of sending DIO messages by the fixed node in order to introduce itself and to be selected as the parent node by the mobile nodes. The mobile nodes did not modify their timer, but this is not suitable for its neighbor nodes to be aware of their current speed in case it changes .The related works showed there was a need for a protocol that supports mobility, they worked on that but this led to an increase in delay and overhead in the network. In this research, new control message was proposed to make the nodes aware of parent node movement to take into account the appropriate time for changing the parent node without waiting for the timer specified in standard RPL protocol.
Table 1: Related works
Fig. 2: ICMPv6 message format [16]
HERE Base object
HERE Base object is proposed to contain the following fields shown in Figure 3:
1- Flags: 8 bits. only 2 bits are reserved for S and H. the 6 bits remaining unused in the Flags field and reserved for flags. The field must be set to zero by the sender and must be ignored by the receiver.
(STOP) S: the ‘S’ flag indicates that the mobile node has stopped and sends a HERE message to its child nodes.
(LISTEN) L: the ‘H’ flag indicates that the node has heard Here message that is sent by mobile nodes and it is still within its radio range even after its movement, so the mobile node does not need to find a new parent node. However, if it moves and no such message arrives, the mobile node needs to find a new parent node as soon as possible to reduce the delay caused by separation resulted by moving the mobile modes when they change their place.
The values of all flag fields remain zero when a message is sent by the mobile node to its parent and child nodes if it moves to let them know.
(0,0) I MOVE TO HERE.
(0,1) LISTEN I’M HERE
(1,0) I STOP HERE.
(1,1) Invalid state.
Fig. 3: HERE Base object
2- Control Message Option Format [16]:
No type of options was proposed, so padding will be used in proposed message. The general form of option contains three parts (Type, Option Length, Option Data). For padding option, the fields will be as following:
The Pad1: option is used to add an octet of zeros for alignment. it has neither option length nor option data. The value of this type is 0x00. Pad1 is shown in Figure 4.
Fig. 4: Pad1
The PadN: option is used to add two or more octets of zeros. The value of this type is 0x01. PadN is shown in Figure 5.
Fig. 5: PadN
Proposed protocol rules
A mobile node must reconnect with a parent node within 5 seconds after it stops moving [17], so the following rules is suggested for proposed protocol:
The main purpose of this paper is to maintain the networks from disconnection for long time although of moving nodes, and to less changing parent node, so we make the node stay connected with the parent node although of its movement while it still in its radio range. The proposed control message is sent to inform the node of the change in the location of the parent node, which maintains communication between nodes by searching for the parent node immediately if it moves. The advantages of the proposed message compared to previous works that it is more suitable for LLN networks because it is sent only when node changes his location so it does not increase the overhead in the network.
Fig. 6: The mechanism of the proposed protocol
Fig. 7: Case study1
Figure 7 shows case study where all messages are received correctly despite of the mobile node movement. The parent and child nodes are still in the mobile node’s radio range.
Figure 8 shows another case study where the mobile node was moving, which led the child node to exit outside the mobile node radio range, so it did not receive a HERE or STOP message. After that, it will search for a new parent node. When the mobile node stops, the parent node is out of range, so it does not receive a LISTEN message and it will search for a new parent node by sending DIS messages to its neighbours.
Fig. 8: Case study2
Protocol Performance Evaluation
In this paper, the proposed protocol was evaluated using the Cooja emulator [18] that supports IoT and all its protocols. Cooja is used because it is an emulator, not a simulator, meaning that its performance is closer to reality because it is running real devices in the network, which makes the results we get more
accurate and simulating reality. This emulator runs on Contiki OS which is open source, multitasking and designed specifically for constrained memory. It supports a wide range of low-power wireless devices, like a Z1 chip or sky mote, etc.
Performance metrics [13]
The proposed protocol was evaluated in terms of PDR, power consumption, overhead, and end-to-end delay. The calculations are as follows:
Simulation Results and Analysis
This section presents a performance analysis of proposed protocols compared to protocols MARPL, FL-RPL, and ARMOR. The networks in the simulation were built using Cooja program, where the simulation parameters were adopted according to previous studies that were compared with. The research [19] suggested the MARPL protocol, which adopted the idea of detecting node movement through the value of RSSI and determining the availability of the neighboring node. If the node receives a DIO message, it updates the Neighbor Variability metric, otherwise, if it receives DAO or DIS control messages, it reduces the time interval between each transmission of DIO messages, which increases their transmission rate and thus more overhead. In the simulation, a 300*300 m2 area was considered with 50 mobile nodes at a maximum speed of 3 m/s with a different number of root nodes (1,2,3). The results in Figure 9 show the value of the packet delivery rate which increases when the number of roots nodes increases.
Fig. 9: Packet Delivery Ratio vs num of roots
By comparing the MARPL simulation results with the proposed protocol, we notice the superiority of the proposed protocol due to its ability to support mobile nodes by making the parent and child nodes of the mobile node aware of its movement via the proposed control message (HERE). MARPL increases the ratio of sending control messages, which causes more overhead on the network and increases collisions because it sends DIO messages to all neighbors, while the HERE control messages are sent only to the parent and child nodes that need this information. Average end-to-end delay: The result shown in Figure 10. Considering that MARPL relied on calculating the value of node availability through the RSSI, this leads to recalculating the value for all nodes every time. In the proposed protocol, even if the node moves and changes its location, it makes sure that the parent node is still within its radio range in order to reduce repeating the search process for a new parent node, and that reduces the power consumption, collision, and delay.
Fig.10: Average End to End delay vs num of roots
FL-RPL [13] is modified the RPL using fuzzy logic with several parameters. The simulation was implemented with an area of 10000^m2, 9 mobile nodes and (15,20,25,30) fixed nodes, the simulation results showed that the value of the packet delivery rate is close when comparing the proposed protocol with the FL-RPL protocol, where the proposed protocol exceeded 3% (Figure 11). The proposed protocol outperforms FL-RPL through reducing the delay by about half because the FL-RPL protocol performs many operations every time a node receives a DIO message, (see Figure 12). The routing metrics are given as input of fuzzy inference system to obtain a confidence score of the node and recalculate the mobility time. Therefore, these steps cause more delay and an increase in power consumption. Figure 13 shows that the proposed protocol reduced energy consumption, especially when the number of mobile nodes was more than fixed ones.
Fig. 11: Packet Delivery Ratio vs num of fixed nodes
Fig. 12: Delay vs num of fixed nodes
ARMOR [15], the research proposed a new parameter TTR to select the best parent node that will stay the longest within the radio range. TTR is calculated based on the node’s speed and position, and it is added to the DIO message. In this paper, a new timer was added to increase the rate of sending DIO messages by the fixed node in order to introduce itself and to be selected as the parent node by the mobile nodes. The mobile nodes did not modify their timer, but this is not suitable for its neighbor nodes to be aware of their current speed in case it changes. The simulation was implemented with an area of 10000^m2, 20 nodes(10 static nodes and 10 mobile nodes) at a speed of 0.5 to 1.5m/s, and one root node. Another scenario was with 40 nodes (20 static nodes and 20 mobile nodes).
Fig. 13: Power Consumption vs num of fixed nodes
The simulation results showed that the packet delivery rate of the proposed protocol is 10% higher than ARMOR (Figure 14) because it supports mobile nodes by making them directly aware of the state of the parent node connected to them. If it becomes out of radio range, it will search for a new parent node.
Fig. 14: Packet Delivery Ratio vs num of all nodes (mobile and fixed)
The routing load of the ARMOR protocol increased because it modified the timer algorithm for static nodes which made them send more control messages, so the mobile nodes are aware and communicate with them.
Fig. 15: overhead vs num of all nodes (mobile and fixed)
The proposed protocol did not increase the rate of sending control messages (Figure 15), so it was less routing load. It relied only on a suggested control message sent by the mobile node to its parent and child nodes when it moves. The power consumption of the ARMOR protocol is higher than the proposed protocol (Figure 16) because it sends more control messages.
Fig. 16: Power consumption vs num of all nodes (mobile and fixed)
Discussion
The research shows the need to support mobile nodes in IoT networks. The proposed work helped to achieve this and reduce the impact caused by the nodes when they move within the network. From the simulation results, we observed that the proposed protocol improved the performance of the RPL protocol. It increased the packet delivery ratio because it made the parent and child nodes of the mobile node aware of its state. So they search for a new parent node immediately when the node moves away without waiting to expire the timer of the trickle algorithm, so it decreased the delay .The proposed protocol characterized that it doesn’t send control messages periodically because this is not suitable for the nature of LLN networks. It minimized overhead because it maintained routing adjacency, focused on links that are used by the mobile node, and did not depend on broadcasting the proposed control message to all neighboring nodes to be more suitable for this type of network because this decreased the power consumption. Therefore, the proposed protocol helps to spread the devices that support mobility (smart watches, smart vacuum cleaners) in IoT networks without impact on the network.
Conclusions:
In this research, a new mechanism is proposed to discover disconnection in the network and makes a node reconnect as soon as possible. This disconnection results from movement of the nodes. Our goal is to ensure that the protocol is lightweight while working to support mobility, because many related studies led to increased the overhead, and others depended on discovering movement of nodes through the value of the signal strength RSSI, which is affected by interference and barriers. The new control message ‘HERE’ is proposed to be sent by the node when it moves to both the parent node and its children. If the parent node receives this message, it will respond by LISTEN message, but if it does not receive LISTEN message, it will search for a parent node. If a node stops moving, it sends a STOP message to notify its child nodes. These operations were set with a timer under the standard times for this type of network. The results showed the superiority of the proposed protocol over previous studies because it helped to reconnect the nodes in the network quickly, which increases the packet delivery rate and reduces the delay caused by disconnections in the network when nodes move. Also, it did not depend on increasing the rate of sending control messages which causes an increase in the network overhead .As this paper focuses on supporting the mobility in LLN networks, our future work is to propose a method to determine the type of device (fixed / mobile) and suggest a mechanism for detecting the movement of nodes in real-time within the network and changing its parent node to more appropriate node, so that it reduces the number of changes the parent node .In addition, we will work on enhancing network stability depending on additional parameters to choose the parent node in the network. This research contributes to increase IoT spreading in many applications in several areas, such as parking system, smart home, health care, etc. Which contain mobile nodes without affecting network performance.
Introduction
Many researchers are concerned with studying the behavior of concrete under the influence of compression loads. Then giving proposals for mathematical models through a mathematical extrapolation that explains the material behavior, which is determined by the relation between stress and strain (σ,ε). However, most of the proposed mathematical equations depend mainly on the results of the experimental tests which could be similar in the general shape with variation in the terms of its application, while depending on the mechanical, chemical, and physical properties of the various particles of concrete. In comparison with the studies that were carried out on concrete containing chemical and filler additives, such as hydraulic, pozzolanic materials, and chemical additives such as plasticizers [1], the topic of SCC self-compacting concrete containing improved materials and the effect of these materials on the molecular structure of the material is considered an important one for research. This is due to the effectiveness and wide spread of this type of modern concrete SCC in many engineering applications. Mohammed H M presented an experimental investigation on the stress-strain behavior of normal [2] and high-strength self-compacting concrete, with two different maximum aggregate sizes. The results show that the ascending parts of the stress-strain curves become steeper as the compressive strength increases, and maximum aggregate size decreases. Jianjie Yu studied the changing regularity of rubber particles’ impact on self-compacting concrete deformation performance [3]. The results show that the rubber particles in self-compacting concrete is more uniform distribution, compared with the reference group. In addition, Selvi K presented an experimental investigation on the modulus of elasticity of self-compacting concrete, involving various flying ash proportions [4], where the stress-strain relationship was studied for the M20 concrete mix. All previous research has studied self-compacting concrete containing fine filler additives, such as flying ash [5]. That gives the concrete distinctive fresh and hardened properties.
This paper will present the production of self-compacting concrete SCC clear of fine fillers by using cement as a fine material that is available and representative of those additives’ fillers.
Materials and Methods
Many previous studies and research have discussed the performance of concrete and described its behavior using the stress-strain curves (σ,ε). This expresses the mechanical behavior of the material [6], throughout deducing a formula that enables us to analyze the behavior of concrete mathematically. In 1951, HOGNESTAD proposed mathematical formula (equations 1 and 2), this enables us to describe the relationship between stress and strain in the ascending, and descending parts of curves [7] for traditional concrete. Figure /1/. So, stress f_c is calculated as a function of the relative strain $ (\frac{\varepsilon_c}{\varepsilon_{co}}) $.
$ f_{c,1}=0.85{f\prime}_c\left[2\frac{\varepsilon_c}{\varepsilon_{co}}-{(\frac{\varepsilon_c}{\varepsilon_{co}})}^2\right]\ \ \ \ \ \ \ \ \ \ \ \ \ \ \ \ \ \ \ \ \ \ \ \ 0>\varepsilon_c>\varepsilon_{co} $ (1)
$ f_{c,2}=0.85{f\prime}_c\left[1-{0.15(\frac{\varepsilon_c-\varepsilon_{co}}{\varepsilon_{cu}-\varepsilon_{co}})}^2\right]\ \ \ \ \ \ \ \ \ \ \ \ \varepsilon_{co}>\varepsilon_c>\varepsilon_{cu} $ (2)
$ f_{c,1} $: the stress of the concrete in the ascending part MPa.
$ f_{c,2} $: the stress of the concrete in the descending part MPa.
$ {f\prime}_c $: the maximum compression strength of the concrete MPa.
$ \varepsilon_{co} $: the strain corresponding to the maximum compression strength of the concrete.
$ \varepsilon_{cu} $: the critical strain of the concrete.
$ \varepsilon_c $: the strain of the concrete.
Fig. 1. HOGNESTAD stress-strain curve
KENT and PARK also presented, in 1971, a proposal for a stress-strain curve model Equation 3 for the confined and unconfined concrete with the descending part only [8]. Figure /2/. So, the stress fc is calculated as a function of the strain corresponding to the maximum strain εco, and the critical strain
$ f_c={f\prime}_c\left[1-\frac{0.5}{\varepsilon_{50u}-\varepsilon_{co}}(\varepsilon_c-\varepsilon_{co})\right]\ \ \ \ \ \ \ \ \ \ \ \ \ \varepsilon_{co}>\varepsilon_c>\varepsilon_{cu} $ (3)
$ \varepsilon_{50u}=\frac{3+0.29{f^\prime}_c}{145{f^\prime}_c-1000}{f^\prime}_c(Mpa) $
Fig. 2. KENT and PARK stress-strain curve
Also, in 1973 POPOVICS presented mathematical formulas. Equation /4/ describing the stress-strain relation of concrete [9]. It was considered that the relative stress as a function of the relative strain according to the following:
$ \frac{f_c}{{f\prime}_c}=\left[\frac{n\frac{\varepsilon_c}{\varepsilon_{co}}}{\left(n-1\right)+{(\frac{\varepsilon_c}{\varepsilon_{co}})}^n}\right] $ (4)
$ n=0.058.{f\prime}_c+1 $
He also presented a mathematical formula. Equation 5. in order to calculate the strain of concrete as a function of the maximum compression strength in the concrete:
$ \varepsilon_{co}=\frac{2{f\prime}_c}{12500+450{f\prime}_c} $ (5)
As for CARREIRA, he followed what POPOVICS had reached and developed a mathematical formula. Equation /6/ for calculating stress as a function of strain [3], where he took the effect of the concrete elasticity factor into account. As follows:
$ \frac{f_c}{{f\prime}_c}=\left[\frac{R(\frac{\varepsilon_c}{\varepsilon_{co}})}{\left(R-1\right)+{(\frac{\varepsilon_c}{\varepsilon_{co}})}^R}\right] $ (6)
$ R=\frac{E_c}{E_c-E_O} $
Where:
$ E_c=5000.\sqrt{{f\prime}_c}\ Mpa $
$ E_O=\frac{{f\prime}_c}{\varepsilon_{co}} $
$ ???: is the strain at the maximum compressive strength of confined concrete ?′?. $
The European Code EN 1992-1-1 also presented a mathematical formula. Equation /7/describes the stress-strain behavior of concrete [4] as a function of the relative strain $ \frac{\varepsilon_c}{\varepsilon_{co}}, $, and related to the elasticity factor of the material. According to the following:
$ \frac{f_c}{{f\prime}_c}=\left[\frac{k\eta-\eta^2}{1+(k-2)\eta}\right] $
$ \eta=\frac{\varepsilon_c}{\varepsilon_{co}} $
$ k={1.05.E}_c\frac{\varepsilon_{co}}{{f\prime}_c} $
SCOPE OF WORK
The Fresh Properties of Self-Compacting Concrete
Three mixtures of self-compacting concrete SCC were produced, in addition to the reference mixture, in different cement quantities [11]. The proportions of materials for the mixes are given in Table 1.
Table 1. The proportions of materials
Mixture | Cement quantity (kg/m3) | W/C | Superplasticizer (%) | Coarse Aggregates (kg/m3) | Fine Aggregates (kg/m3) |
SCC | 550
500 450 |
0.390 | 2.0
2.5 2.5 |
625 | 1000 |
The tests of the fresh properties of SCC were conducted [14]. They include J-ring Test, Slump Flow (SF) and Test, Visual Stability Index (VSI), and Segregation Test (SR). As shown in Figure 3.
Fig. 3. SCC-HRW Fresh Properties Tests
The rules for adopting the ratio of superplasticizer depend on the technical specification and guidelines for using this type of material, where the recommended ratio range (from 0.5 to 2.5) % of cement weight. In the lab tests, the initial ratio was 1% and then increased till it achieved the required operability in each one of the mixes.
It was found that the mixture with a proportion of cement quantity (550 kg/m3) containing plasticizer coded as HRW gives the preferred properties the operability, Table /2/. The mixture with (450 kg/m3) cement quantity does not give any of the required properties for this type of concrete.
Table 2. SCC fresh properties:
Mixture | Cement quantity (kg/m3) | W/C | Superplasticizer (%) | Slump Flow SF (cm) | (sec) | J-ring (DJ %) | Segregation Test (SR%) | |
SCC-HRW-550 | 550 | 0.39 | 2.0 | 58 | 5.40 | 86 | 6.42 | |
SCC-HRW-500 | 500 | 0.39 | 2.5 | 52 | 4.86 | 96 | 4.06 | |
SCC-HRW-450 | 450 | 0.39 | 2.5 | – | – | – | – | |
Allowed | 55-65 | – | ≥80% | ≤15% |
Strength of SCC Samples on the Axial Compression
The laboratory tests result of SCC cylindrical samples produced locally at uni-axial compression [13] show the contribution of the plasticizer by reducing the ratio W/C up to 13%. Thus, there is an improvement in the SCC concrete in terms of strength and strain behavior. Figure 4.
Fig. 4. Fracture Test of SCC Cylindrical Samples
According to laboratory tests, an increase in the strength of concrete containing plasticizer HRW (high reduction water) by 2% of the cement weight up to 12% has been noted, compared to the reference sample, which has the same proportions of materials but without a plasticizer.
The plasticizer in all concrete mixtures contributed to an increase in the operability and a decrease in the W/C ratio. The percentage up in the strength was due to the quantity of cement used, the type of plasticizer, the percentage of plasticizer, and the W/C ratio [12]. The decrease in the compression strength was observed by using a lower quantity of cement with a higher percentage of plasticizer. This can be explained by the change in the molecular structure of SCC mixtures when the ratio W/C is stable. So, it is better to use a higher quantity of cement for a lower plasticizer percentage, and stability by a ratio of W/C.
Since the concrete molecular structure is made up of two components, aggregates and paste. Aggregates are generally classified into two groups, fine and coarse, and occupy about 70% of the mix volume. The paste is composed of cement, water, and entrained air and ordinarily constitutes 30% of the remaining volume.
The major change in the molecular structure of the mixture under the influence of the superplasticizer is on the cement paste, which affects one component or more of the three mentioned.
Table 3. below shows the increase in the cylindrical compression strength depending on the cement quantity, the percentage of plasticizer, and W/C ratio.
Table 3. Compressive strength of SCC mixtures
Mixture | Cement quantity (kg/m3) | W/C | Superplasticizer (%) | Compression Strength (MPa) | Strength Increase Ratio (%) |
RS-550 | 550 | 0.448 | – | 33,572 | – |
SCC-HRW-550 | 550 | 0.39 | 2 | 37.616 | 12 |
RS-500 | 500 | 0.448 | – | 27.717 | – |
SCC-HRW-500 | 500 | 0.39 | 2.5 | 28.249 | 1.9 |
RS-450 | 450 | 0.448 | – | 24.680 | – |
SCC-HRW-450 | 450 | 0.39 | 2.5 | 24.890 | 0.01 |
In the mixes with cement quantities 450 kg/m3, it is noted that the compression strength takes the same result with or without a plasticizer. This can explain the excess dose of the plasticizer causes additional dispersion and scattering of the cement granules, due to the repulsion of the negative charges that envelop them. Thus, there was a lack of bonding of the cement granules. Another reason that could occur was an increase in the additional air to the mixture.
Figure 5. shows the relation between the strength and the plasticizer percentage used, according to the quantity of cement in the SCC samples.
Fig. 5. SCC Strength and Plasticizer Percentage Relation
According to the previous data of the tests, and by searching for the mathematical equation that is closest and most representative of the relation between the strength of SCC and cement quantity and by using Curve Expert1.4 software program for processing curves, many mathematical curves were obtained. Figure 6. shows the closest curves to the study case with correlation coefficient R.
Fig. 6. The Closest Mathematical Curves
The above three mathematical graphic curves are closest to our experimental tests. It is clear from Figure 6 that the model known as the Rational Function gives the closest model with the best correlation factor R closer to 1. Equation 8, the realistic behaviour of the SCC, and the most expressive of the relation between the cement quantity and the strength of the self-compacting concrete, on the axial compression for different plasticizer ratios. While the other curves show a continuous sharp decline in the strength between the defined points, and an infinitely increasing of strength. This contradicts the relation between the concrete strength and the cement grade.
The Equation of Rational Function is given as:
$ y=\frac{a+bx}{1+cx+dx^2} $ (8)
y: Compression Strength of SCC (MPa).
x: Cement Grade (kg/m3).
a,b,c,: Equation constants, substituting the values of the constants into Equation (1). Founded that the equation takes the following form (9):
$ y=\frac{-45572.93+48.36x}{1-7.74x+0.0124x^2} $
Proposed Model of the Stress-Strain Formula for the Self-Compacting Concrete
The chemical materials in the self-compacting concrete led to a change in the molecular composition of the material. Thus, a change in its mechanical properties affects the behaviour of the concrete in fresh and hardened cases.
Through the application and analysis of previous models of the relation between stress-strain on SCC prepared in the lab, it was found that models can express the behaviour of concrete SCC in varying proportions. As for, the convergence was clear with the lab model, in the ascending part of the curve until reaching the ultimate stress Figure 7. But the difference was in the descending part of the curve. Therefore, it was interesting to search for a mathematical model that describing and expressing the closest behaviour for SCC in ascending and descending parts of the curve.
To understand the behaviour of hardened concrete, and through accurate processing of the numerical results of the fracture tests on the uni-axial compression of cylindrical samples. Models were achieved to describe the stress-strain occurred under the influence of the uni-axial compressive force.
To obtain a general unconditional formula, unrestricted in terms of the quantity of cement and the proportion of plasticizer used in the mixture. Data were processed and converted into non-dimensional relative values allowing the conversion of the data from the specific case into the general state of the tested concrete.
Fig. 7. SCC and Reference Stress-Strain Curves
It was started from the same premise of previous studies and models in mathematical treatment by converting stress f_c into relative stress by dividing it by $ {f\prime}_c $, and the measured strain \varepsilon_c into relative strain by dividing it by $ \varepsilon_{co} $. So, the values of the mathematical treatment for stress and strain, are according to the following:
$ \frac{f_c}{{f\prime}_c} $: nominal relative stress, where: $ {f\prime}_c $: the maximum cylindrical strength of the concrete.
$ \frac{\varepsilon_c}{\varepsilon_{co}} $: nominal relative strain, where:$ \varepsilon_{co} $: strain corresponding to the maximum stress of the concrete.
To find a mathematical model that expresses the behavior of this type of concrete, the results of lab experiments of samples produced of SCC were entered at several cement quantities, and by defining it as nominal relative values $ \frac{f_c}{{f\prime}_c} $, $ \frac{\varepsilon_c}{\varepsilon_{co}} $ to obtain the optimal mathematical curve for this case, which gives the best correlation coefficient R and the smallest standard error.
The treatment showed that the most appropriate mathematical formula for the curve, in this case, is the Rational Function form, Equation 10. which is the general form:
$ y=\frac{a+bx}{1+cx+dx^2} $ (10)
y: the compressive strength in the concrete.
x: the strain in the concrete.
a, b, c, d equation constants.
The equation shown above and in its general form does not achieve the conditions of the model we are looking for, in terms of stress and relative strain. Figure 8 below shows the experimental and the equation curves form:
Fig. 8. SCC and Rational Function Equation Stress-Strain Curves
Starting from the performance form of the SCC expressed by the stress-strain curve. The equation was reformulated of the closed model in its general form, using the values of relative stress and strain. So that form of Equation 11. and Equation 12. becomes according to the following:
$ \frac{f_c}{{f\prime}_c}=\frac{a+b(\frac{\varepsilon_c}{\varepsilon_{co}})}{1+c(\frac{\varepsilon_c}{\varepsilon_{co}})+d{(\frac{\varepsilon_c}{\varepsilon_{co}})}^2} $ (11)
$ f_{cnorm}=\frac{a+b(\varepsilon_{cnorm})}{1+c(\varepsilon_{cnorm})+d{(\varepsilon_{cnorm})}^2} $ (12)
$ f_{cnorm}=\frac{f_c}{{f\prime}_c} $ nominal relative stress
$ \varepsilon_{cnorm}=\frac{\varepsilon_c}{\varepsilon_{co}} $ nominal relative strain.
Therefore, the proposed general mathematical formula could be expressed with the values of constant b in the ascending and descending parts of the curve.
Through the observation of the two formulas, there is an incomplete similarity in general. However. this similarity can be complete when the value of the constants as below:
n=b=2
The proposed and POPOVICS formulas take the following form 21:
$ \frac{f_c}{{f\prime}_c}=\frac{2\frac{\varepsilon_c}{\varepsilon_{co}}}{1+{(\frac{\varepsilon_c}{\varepsilon_{co}})}^2} $ (21)
It can also verify the conformity of the behaviour proposed in Eq.21 with the actual samples graphically. Below are the stress-strain curves of the proposed formula and the experimental sample. Figure .10:
Fig. 10. Stress-Strain Curves for The SCC Proposed Mathematical Formula
It has been found that the mathematical equation 21 in which the POPOVICS formula and the proposed formula are similar, gives graphic curves describing the behavior of local SCC with a convergence of up to 90%.
CONCLUSION
This paper aimed to understand the mechanical behavior of SCC samples. Through laboratory verification and mathematical analysis. The key findings of this study are presented below:
The possibility to produce an acceptable Self-Compacting Concrete SCC from Syrian raw materials.
Outputting a mathematical model that enables us to directly calculate the behavior of all types of SCC samples, and to represent it graphically.
The proposed mathematical model describes the behavior of concrete up to 90% approximate, whatever the quantity of cement.
Introduction
Humanity is struggling to create chemical-free, GMO-free, hormone-free, antibiotic-free, safely clean, high-protein nutritional food with low-to-neutral carbon and water footprints at a reasonable price (1). Therefore, improving the sustainability of dairy operations is a current key goal in the dairy sector, and one critical task to increase sustainability is to reduce environmental consequences from dairy production (2). Hence, when evaluating feedstuffs to establish their nutritional contents and inclusion rates in dairy rations, the environmental impact, as well as production responses, should be taken into account. In fact, tremendous effort was put into finding new livestock dietary formulas to tackle the above-enlisted challenges around the globe (3,4). Cattles, and particularly dairy production systems, significantly contribute to green house gas (GHG) emissions and global warming mostly through the creation of methane (CH4) (5). In fact, methane is the largest contributor to global warming from the dairy sector, with a 28 times higher impact compared to carbon dioxide (CO2) over a hundred-year period (6). Therefore, the transformation of our production systems with a particular focus on lowering GHG emissions has gained priority (7). In this context, lessening environmental impacts from dairy production is one critical task to improve sustainability of dairy operations. Thus, the environmental impact, as well as production responses, should be considered when evaluating feedstuffs and determining their nutritional values and inclusion rates in dairy rations. Yan et al. (8), demonstrated that one attribute of energy-efficient cows is that less methane is produced relative to the amount of energy consumed or milk produced. Other studies have shown that breeding for cattle with high feed efficiency may also result in decreased daily enteric methane generation, due to the strong genetic and phenotypic association between daily methane output and residual feed intake (9,10). On another side, profitability will rise due to improved feed efficiency because feed expenses are the main expense on dairy farms. Feed efficiency are expressed in various ways, including feed conversion efficiency (milk output over feed intake). In theory, improved feed efficiency decreases daily methane production due to a lower methane per kilogram of dry matter intake (DMI) at a given production level (11), while decreased methane production (e.g., due to nutritional strategies) does not necessarily improve feed efficiency. However, experimental data are inconsistent on the link between residual feed intake (RFI) and methane emission, while research has primarily focused on beef cattle (12,13) rather than on lactating dairy cows (14) .Recent developments in livestock nutrition have primarily concentrated on three areas: improving our understanding of the nutritional needs of livestock, identifying the supply and availability of nutrients in feed ingredients, and developing the least expensive diets that effectively combine nutrient requirements and nutrient supply (15-17). In line with this strategy, the main objectives of our applied research are to reverse the livestock conventional common practices from high multi-dimensional polluter into a low polluter sector with a low water footprint; improving the quality of protein and fatty acids profiles for animal and human better health and well-being, and reducing the overall cost compared to organic practices. To achieve the objectives of this project, nearly a decade worth of applied research resulted in the development of a new balanced feed intake diet composed of a clean fresh sprouted highly nutritional mix, namely Mahjoub Feedstock Diet (MFD), produced in soil-less vertical farming in a controlled environment. Neutral carbon footprint (NCF) and low water footprint (LWF) resulted into chemicals-free, hormones and genetically modified (GMO) free husbandry practice at a local facility in Damascus, Syria. This study is a continuation of our applied research, and it is worth mentioning that it is the first to evaluate whether manure nutrients, NH3 emissions and milk quality were affected by feeding cows with MFD.
Material and Methods
Mahjoub’s feedstock diet
Mahjoub feedstock (MFD) diet is an innovation primed for livestock feed. This innovation in feed ingredients covers efficiency, profitability, environmental footprint, animal health, and welfare. All chemical and physical analyses were conducted in Cumberland Valley Analytical Services (USA) according to standard and accredited protocols. MFD, with Mahjoob’s Intellectual Properties, is a clean fresh sprouted diet produced in a controlled-environment vertical farming powered entirely by clean renewable energy resulting in a neutral carbon footprint and a very low water footprint at a local facility in Damascus, Syria.
Animals and Treatments
We conducted our experiments in a randomized complete block design. We fed four “Holstein” cows (average 506 ± 100 kg) on MFD diet over a period of two years. For manure comparison only, we collected fresh manure samples from cows fed a local common basal diet-containing soybean as a control (CON) and compared its chemical and physical composition to fresh manure collected from cows fed on MFD. On the other hand, we compared the composition of milk-fat produced by cows fed on MFD to a world-renowned brand butter fat sample. MFD was prepared once a day in the morning and fed to cows four times/24h, namely at 7am, 13:00, 17:00 and 20:00. Notably, cows were free-stall most of the day with access to outdoor and fed through designated feeding box. Finally, cow bedding was made of dried odour-less dried manure. Sample Collection and Measurement – milk production and composition. Cows were milked 2x daily with milk yields average around the year approximately 25 liter/day. Milk samples were obtained by automated milking machine and collected into clean and steamed containers, with measurements performed within one hour at the laboratories of the National Commission for Biotechnology (NCBT), Damascus, Syria. GC-MS standard protocols were used for fatty acid analysis (Thermo Scientific, USA) while amino acid analysis was performed by amino acid analyser (Agilent, USA). All chemical and colorometric assays for total protein and manure analysis were performed using standard protocols at NCBT.
Results
Feed Diet Analysis
Chemical analyses were performed on Mahjoub’s feedstock diet (MFD) (Table 1).
Effect of Mahjoub’s feedstock diet on Manure Nutrient Content. The characteristics of the manure samples from cows fed MFD and meal local common diet are shown in Table 2.
Table 2. Characteristics of the manure samples from cows fed Mahjoub’s feedstock diet and meal local common diet
Properties | Unit | Basis | Manure from Cows fed MFD | Manure from Cows fed CON |
Dry matter | wt% | wet | 17.96 | 17.86 |
Volatile solids | wt% | dry | 86.64 | 80.70 |
Ash | wt% | dry | 13.36 | 19.26 |
Carbon | wt% | dry | 50.22 | 46.80 |
Nitrogen | wt% | dry | 1.65 | 2.20 |
C/N | wt% | dry | 30.40 | 21.27 |
Ammonia | wt% | wet | 0.07 | 0.21 |
Fiber | wt% | dry | 53.11 | 49.58 |
Calcium | wt% | dry | 2.34 | 2.07 |
Phosphorus | wt% | dry | 0.17 | 0.26 |
Potassium | wt% | dry | 0.67 | 0.72 |
Sodium | wt% | dry | 0.17 | 0.14 |
Chloride | wt% | dry | 0.21 | 0.83 |
Iron | wt% | dry | 0.011 | 0.012 |
Electrical conductivity | μS/cm | – | 180 | 470 |
PH | – | – | 7.7 | 7.25 |
MFD = Mahjoub’s feedstock diet; CONT = fed basal diet containing soybean (meal local common diet)
Analysis of Protein and Fat in Milk Products
We compared the amino acid (AA) profile between MFD and cow milk to explore the possible cow/rumen conversion of AA in vivo (Fig 1). To study the effect of MFD on fat profile, we compared the fat contents in milk-fat from MFD-fed cows to a globally well-known butter brand (Fig 2).
Fig. 1. Comparison of amino acid concentrations between the original MFD (g/day of feed intake) and cow milk (g/day produced) fed on MFD.
Fig. 2. Fatty acids composition in average milk fat from cows fed on MFD in comparison with a renowned fat brand. USF: unsaturated fat, SF: saturated fat, TF: trans-fat, w9: omega 9, w6: omega 6, w3: omega 3, PUSF: polyunsaturated fat, MUSF: monounsaturated fat, CLA: conjugated linoleic acid.
Discussion
MFD composition
Results showed several privileged characteristics of MFD when compared to conventional cow feed diets (18) (Table 1) explicitly; high protein, low fat, low volatile fatty acids (VFA), high soluble protein SP/crude protein CP, neutral dietary cation-anion difference (DCAD), low starch, high acid detergent fibers (ADF), and near neutral pH. This composition may reflect the sprouted non-stiff format of MFD and makes it a unique high protein diet, which might positively reflect on cow health.
Manure Composition
Despite the fact that manure is a valuable fertilizer, it has the potential to harm the environment in terms of odor, air, soil, and water quality (19). As various types of gases (e.g., NH3, greenhouse gases, and H2S) are created from manure via microbial fermentation or chemical changes, farm odor and a reduction in air quality at stalls and during manure storage before application to the field may occur (20). It is worth knowing that the amount of gas generated by manure is determined by both internal and external factors. External influences include chemical forms of nutrients and nutrient concentrations, temperature, humidity, wind, bedding, manure storage system, and so on. Internal factors may include the cow genetic makeup and the microflora residing in their intestines.
In our study, we assessed changes in manure characteristics as well as potential gas emissions from manure. In fact, feeding the cows on MFD diet tended to increase manure pH compared to controls (7.7 vs.7.26) (Table 1).
The content of organic matters was greater for MFD versus CONT, without a difference in dry matter (DM). It is worth noting that manure nitrogen content was lower for MFD versus CONT (1.65 vs. 2.2 %), and this could be a factor that potentially lowers NH3 emissions from manure because manure N, in the form of urea, contributes to NH3 emitted from manure (21,22). Our results showed that the cumulative ammonia production for MFD was lower than its production from CONT by a factor of three (0.07 vs. 0.21 %). The degree of the decrease in NH3 emissions by MFD in this study is similar to the decrease observed when feeding cows on a
low-protein diet (23). Thus, our data demonstrate that the NH3 -emitting potential of manure can be reduced using MFD without decreasing dietary protein content, as the high protein content of MFD was not associated with high manure nitrogen, as one would expect.
Table 1. Chemical analysis of Mahjoub’s feedstock diet
Properties | Unit | Basis | MFD |
Dry matter | wt% | wet | 24.9 |
Ash | wt% | dry | 5.56 |
Crude protein | wt% | dry | 28.2 |
Soluble Protein | wt% | dry | 17.6 |
Crude fat (fat ether extract) | wt% | dry | 2.83 |
Starch | wt% | dry | 11.8 |
Starch | wt% | NFC | 44.0 |
Soluble Fiber | wt% | dry | 9.51 |
Soluble Fiber | wt% | NFC | 35.4 |
Volatile fatty acids | wt% | dry | 5.58 |
Lactic Acid | wt% | dry | 2.20 |
Lactic Acid | wt% | VFA | 39.4 |
Acetic Acid | wt% | dry | 3.38 |
Propionic Acid | wt% | dry | 0.19 |
Ammonia | wt% | dry | 17.3 |
Lignin | wt% | dry | 4.24 |
Soluble Fiber | wt% | dry | 9.51 |
Non Fiber Carbohydrates | wt% | dry | 26.8 |
Non-Structural Carbohydrates | wt% | dry | 13.4 |
Acid detergent fibres | wt% | dry | 25.1 |
Total digestible nutrients. | wt% | dry | 68.4 |
PH | 6.68 |
MFD= Mahjoub’s feedstock diet; NFC= non-fiber carbohydrate; VFA= volatile fatty acids
The C/N ration in manure from MFD-fed cows was profoundly higher compared to manure from locally fed cows (30.4 vs. 21.27), while total nitrogen was lower (1.65 vs 2.2) and fiber content was close (53.11% vs. 48.58%), compared to manure from locally common fed cow. The profoundly lower electrical conductivity (180 vs. 470 μS/cm) and higher pH (7.7 vs 7.25) in MFD-fed compared to locally fed cows may enhance the applicability of the fresh manure from the former as a proposed soil substrate replacement. Worth to mention, the low ammonia concentration may have resulted in a near-no odour of manure.
Amino Acid Profile
Our results show a major increase in several amino acids upon feeding on MFD, specifically two essential AA (proline and glutamate), and leucine, a non-essential AA. Interestingly, these three previous AAs were proposed to play a main role in regulating and enhancing the immune response in both cows and humans (24,25). In fact, it is well known that amino acids regulate the activation of many immune cells including T and B lymphocytes, natural killer cells and macrophages, in addition to controlling gene expression and the production of antibodies and cytokines (24). Nevertheless, one major finding about MFD-fed cows was the antibiotic-free wellbeing of the four cows in study over the last two years. This wellbeing is supported by physical in addition to biochemical analyses of several cow plasma biomarkers, all of which were continuously within reference ranges throughout the study (data not shown).
Fat Profile
The results showed several excellent features of the MFD on human health and wellbeing (26), including: slightly higher unsaturated fat and lower 6/3 ratio compared to brand fat, a favourable profile in many health compromised situations including heart disease (27,28). More importantly, fat from MFD contained substantially favourable lower trans-fat (TF) in MFD-milk fat compared to the brand fat (0.79 vs 3.13, respectively). In fact, previous research proved a direct link between TF and many diseases including cardiovascular, breast cancer and disorders of nervous system, etc (29). Additionally, MFD-fat contained two fold levels of conjugated linoleic acid (CLA) in comparison to the brand fat (0.62 vs. 0.31, respectively). CLA has several beneficial health effects as it reduces body fat and consequently alleviates the risk for cardiovascular diseases and cancer. In addition, CLA modulates immune and inflammatory responses as well as improves bone mass (30). Finally, both saturated C15 and C17 were markedly higher in MFD-fed cow fat compared to brand (C15; 0.86 vs. 0.40 g/100g fat) and (C17; 0.87 vs. 0.42 g/100g fat), respectively. C15 odd saturated fatty acids are linked to supporting metabolic and heart health, while both C15 and C17 fatty acids are associated with lower risks for cardiovascular diseases and mortality (31,32). Taken together, the MFD-milk fat profile suggest an enhanced human wellbeing.
Conclusion
This study was the first to evaluate whether manure nutrients, NH3 emissions and milk quality were affected by feeding cows with Mahjoub’s feedstock diet. Our results show a major increase in several amino acids in the milk of cows fed with MFD, which we propose to play a main role in regulating and enhancing the immune response in cows. Indeed, this could be supported by the fact that cows fed on MFD were antibiotic-free well-being for many years.
On another hand, our results indicate that the NH3-emitting potential of cow manure were reduced by MFD without decreasing dietary protein content. Hence, a beneficial goal was achieved without jeopardizing the cow immune response relying on adequate protein concentration in the diet .Finally, the low ammonia values in MFD-fed cow manure, low total nitrogen, high fiber compared to local common-fed cow manure, low electrical conductivity and alkaline pH, will enhance the applicability of the fresh manure from MFD-fed cows as a proposed soil substrate replacement and may have resulted in a near-no odour of manur. More studies on the long-term incubation of manure will be necessary to understand H2S emissions during manure storage. In this context, further research is planned and ongoing; our preliminary results show predictable privileged characteristics of MFD on both environment and cow/human wellbeing.
Introduction
Polycystic ovary syndrome (PCOS) is one of the most prevalent conditions affecting women of reproductive age. It affects 6%–20% of premenopausal women globally (1). Ovarian dysfunction and an excess of androgen are the two main symptoms of PCOS. Menstrual abnormalities, hirsutism, obesity, insulin resistance, cardiovascular disease, in addition to emotional symptoms like depression (2) are all common among PCOS patients (3). As a result, it is crucial for the accurate diagnosis and treatment of PCOS. In 1985, Adams et al. (4) discovered that polycystic ovaries have an abnormally high number of follicles, also termed multifollicularity. It was suggested that PCOS be diagnosed when at least two of the three following features were present at an expert meeting in Rotterdam in 2003: (i) oligo- or anovulation, (ii) clinical and/or biochemical hyperandrogenism, or (iii) polycystic ovaries (5). The latter of which can be detected using ultrasonography which offers the highest contribution to the diagnosis of PCOS (6). Early detection of PCOS is important because it can help to manage the symptoms and reduce the risk of long-term health issues. Although ultrasound images have some disadvantages of strong artifacts, noise and high dependence on the experience of doctors, they are still considered as one of the most widely used modalities in medical diagnosis. Many artificial intelligence systems have been developed to help doctors. Convolutional Neural Networks (CNNs) and deep learning have achieved great success in computer vision with its unique advantages (7). Many diseases are diagnosed using different Deep Learning Models (7). Some examples include the detection of COVID-19 using lung ultrasound imagery achieving 89.1% accuracy using InceptionV3 network (8), the use of deep learning architectures for the segmentation of the left ventricle of the heart (9), and the classification of breast lesions in ultrasound images obtaining an accuracy of 90%, sensitivity of 86%, and specificity of 96% by utilizing the GoogLeNet CNN (10). As we can see, deep learning has proved its potential and the vital role it can provide in benefitting and assisting practitioners that use ultrasonography as a tool for diagnosis. This paper is discussing the robustness of deep learning in diagnosing PCOS. Since arificial intelligence (AI) and deep learning algorithms can quickly and reliably assess vast volumes of data, they can be utilized to diagnose PCOS in ultrasound scans. AI and deep learning algorithms are expected to examine ultrasound images to find patterns and traits that are indicative of PCOS in the case of PCOS detection. This can help to increase the speed and accuracy of diagnosis as it can be done more accurately and efficiently than by manual analysis. Furthermore, the application of AI and deep learning in the diagnosis of PCOS can decrease the workload for medical professionals and free them up to concentrate on other responsibilities. Overall, the use of AI and deep learning in the detection of PCOS in ultrasound images has the potential to improve the accuracy, efficiency, and accessibility of healthcare. This was the motive to tackle such an important health issue that affects millions of women worldwide and apply the potential of deep learning to help nullify this crucial problem. Obtaining a viable and correct ultrasound dataset for this task is difficult as the annotation of medical images requires significant professional medical knowledge, which makes the annotation very expensive and rare as well as the ethical issues and sensitivity of such dataset which can pose another problem. Therefore, resorting to a publicly available dataset was chosen to accelerate the work on this project. After observing some of the related work, one publicly available PCOS dataset was utilized in the training of PCONet, a CNN developed by Hosain AK et al. that detects PCOS from ovarian ultrasound images with accuracy of 98.12% on test images as well as fine-tuning InceptionV3 model achieving 96.56% accuracy (11). The PCOS dataset is publicly available on Kaggle (12). Other related work includes Wenqi Lv et al. who utilized image segmentation technique using U-Net on scleral images then a Resnet model was adapted for feature extraction of PCOS achieving classification accuracy of 0.929, and AUC of 0.979 (13). Subrato Bharati et al. used clinical attributes of 541 women in which 177 are infected with PCOS to be utilized in a machine learning model that uses random forest and logistic regression to predict PCOS disease for which the testing accuracy achieved is 91.01% (14). Sakshi Srivastava et al. employed a fine-tuned VGG-16 model to train on their dataset that consists of ultrasound images of the ovaries to detect the presence ovarian cyst with 92.11% accuracy obtained (15). In this paper, the Kaggle dataset highlighted previously is used to conduct training by enhancing the power of transfer learning to train an existing model architecture that is pre-trained on thousands of images in advance. The training on this dataset achieves excellent results. However, after achieving great results and after further inspection on the dataset, it turned out that the publicly available PCOS dataset that was utilized in training the fine-tuned model and that other authors (11) have been using in their research is extremely erroneous and full of misleading information. This will be discussed in details in this paper.
Methodology
Dataset Description
An appropriate dataset is vital for the proper functioning of any deep learning framework.
Thus, the publicly available PCOS ultrasound images available on Kaggle (12) is used. This same dataset is referred to as dataset A in the highlighted paper of Hosain AK et al. (11) mentioned previously and will be referred to as dataset A in this paper as well. A screenshot of the website providing this data is shown in Figure 1 and Figure 2.
Figure 1: Screenshot of the publicly available PCOS dataset on Kaggle consisting of `infected` and `notinfected` ovarian ultrasound images referred to as Dataset A
Figure 2: Statistics of Dataset A show that it is downloaded 301 times out of 2394 views. This means that almost 1 out of every 10 viewers downloads this dataset for utilization in research/projects
The dataset consists of 3856 ultrasound images divided into 2 classes which are labeled as: `infected` and `notinfected`. The latter depicts healthy ovaries and the first indicates the presence of PCOS. These images are partitioned into train and test sets in which 1932 images belong to the test set and the rest belong to the train set. However, the same images seem to be repeated in the test and train directories. Therefore, one of the directories was neglected. Figure 3 depicts a sample of the ultrasound images present in this dataset.
Figure 3: Samples of infected and healthy ovaries in Dataset A
Data Pre-Processing
There are several steps that may be taken to preprocess medical ultrasound images before they are input into a deep learning model for training. It’s important to note that the specific preprocessing steps will depend on the characteristics of the images and the specific requirements of the deep learning model being used. Since the model chosen for this project is the DenseNet201 architecture which is a pre-trained model on the ImageNet dataset, the ovarian ultrasound images that will be the input to this model need to be pre-processed the same way as the images this model was originally trained on. These images vary in size and dimensions therefore they need to be preprocessed so that they are uniform in this aspect. The preprocessing pipeline include:
mean = [0.485, 0.456, 0.406] std = [0.229, 0.224, 0.225]
This is done due to it being mentioned as a necessary pre-process in the documentation (16) of DenseNet201.
Model Description
After pre-processing the data, a classification model is needed. Reviewing related work, most of research done in the medical field that uses deep learning utilizes transfer learning to train on the data through a pre-trained model. In fact, the conclusion of paper (17) mentions that transfer learning is the best available option for training even if the data model is pre-trained on, it is weakly related to data in hand.
Thus, in order to train on this dataset, the DenseNet architecture (18) was chosen. DenseNet is one of the leading architectures used in ultrasonography analysis when conducting transfer learning as table 3 in this paper (19) shows. DenseNet is a variation of the traditional CNN architecture that uses dense blocks, where each layer in a block is connected to every other layer in the block. This allows the network to pass information from earlier layers to later layers more efficiently, alleviate the problem of vanishing gradients in deeper networks, and can improve performance on tasks such as image classification. There are several variations of the DenseNet architecture, such as DenseNet121, DenseNet201, and DenseNet264. Each variation refers to the number of layers it consists of. DenseNet201 is the variation chosen for this project as it has a moderate number of layers and it is not too computationally demanding. DenseNet and many other pre-trained architectures are pre-trained on the ImageNet dataset which is long-standing landmark in computer vision (20). It consists of 1,281,167 training images, 50,000 validation images, and 100,000 test images belonging to 1000 classes. These images consist of a wide variety of scenes, covering a diverse range of categories such as animals, vehicles, household objects, food, clothing, musical instruments, body parts, and much more. A question might arise from this information, why would a model trained on a such unrelated dataset might be used for training on and predicting medical images? In fact, the paper titled “A scoping review of transfer learning research on medical image analysis using ImageNet” (19) discusses this very topic. After inspecting tens of research papers and studies that utilize ImageNet models to train on medical datasets, Author proves that transfer learning of ImageNet models is a viable option to train on medical datasets. The idea behind transfer learning is that although medical datasets are different from non-medical datasets, the low-level features (e.g., straight and curved lines that construct images) are universal to most of the image analysis tasks (21). Therefore, transferred parameters (i.e., weights) may serve as a powerful set of features, which reduce the need for a large dataset as well as the training time and memory cost (21). The structure of DenseNet is shown in figure 4:
Figure 4: An ultrasound image with size (224, 224, 1) as an input to the DenseNet model using its weights and architecture to make a prediction
Model Fine-Tuning
Transfer learning can be utilized to import the DenseNet201 model and fine-tune it to adjust it to according to the dataset. This fine-tuning in this study involves 2 stages:
After fine-tuning the model, the total number of parameters in the model is 18,088,577 in which 1,921 is trainable, and 18,086,656 is non-trainable (frozen).
Picking Loss Function and Optimizer
When working on binary classification in PyTorch, the most common loss function to use is binary cross-entropy loss, also known as log loss. This loss function is appropriate for binary classification problems where the output of the model is a probability, and the goal is to minimize the difference between the predicted probabilities and the true labels. The loss is calculated as:
loss = -(y * log(p) + (1 – y) * log(1 – p))
As for the optimizer, the two most common optimizers used are Adam and Stochastic Gradient Descent (SGD). The latter was the choice for this project as this paper (22) mentions that SGD generally performs better on image classification tasks. The optimizer and the learning rate are closely related, as the optimizer uses the learning rate to determine the step size when making updates to the model parameters. The learning rate opted for is 0.01.
Results:
After conducting the above steps and setting the learning rate to 0.01, the optimizer is set to SGD and training the model is initiated for 15 epochs. Moreover, the model had its pre-trained weights frozen except for its final Linear layer. This method is recommended in the paper (19) for models trained on ImageNet architectures. Exceptional results were achieved with 100% accuracy on the train set and 99.83% on the test set. The following two figures show the loss and accuracy during training in addition to the confusion matrix obtained by the results of the trained model predicting on the test set. Since the results are suspiciously perfect, we decided to investigate more using another dataset.
Figure 5: Results of loss and accuracy during training epochs
Figure 6: Confusion Matrix for Dataset A
Working with a New Dataset
Same experiment using the same parameters was repeated on another dataset: Dataset B. Another publicly available dataset is published by Telkom University Dataverse (23). Figure 7 shows a screenshot of the website providing this data. Dataset B consists of 54 ultrasound images 14 of which are classified as PCOS and the rest are normal. Figure 8 below shows a sample from this dataset.
Figure 7: Screenshot of the publicly available PCOS dataset by Telkom University Dataverse referred to as Dataset B in this paper which is an ovarian ultrasound dataset that is annotated by specialist doctors
Figure 8: Samples of infected and healthy ovaries in Dataset B
However, to keep the two experiments identical and due to the problem of dataset B being relatively small in data number, data augmentation techniques such as random horizontal flipping, random vertical flipping, random brightness alteration, and random rotation are applied using the Python library `imgaug`. Data augmentation increases the number of ultrasound images which improves the training process massively.
Dataset B Results
After training the same model again for 15 epochs on this new dataset and keeping all the hyperparameters fixed, the following results and confusion matrix are obtained:
Figure 9: Results of accuracy and loss during training epochs with the new data for Dataset B
Figure 10: Confusion Matrix for Dataset B
As figure 9 shows, the train accuracy increases steadily until it reaches 83.33% while the test accuracy remains relatively unchanged at 62.92%. The same can be observed for train and test loss where the train loss steadily decreases but not for the test loss. This is a clear indication of overfitting and the inability of the model to generalize well on unseen data. The poor model performance is also confirmed in the confusion matrix where the number of true positives and true negatives is unsatisfactory.
Table 1 exhibits the precision, recall and F1-score for the infected data points in both datasets:
Table 1: Precision, recall, and F1-score for the infected data points in both datasets
Further inspection on the dataset A and after consulting a professional specialist in this medical field, it turned out that the dataset is highly erroneous and misleading. The `notinfected` class which is supposed to represent the healthy ovaries having no sign of PCOS are in fact not images of ovaries at all. Rather, they are ultrasound images of uterus which completely falsify this dataset.
Conclusion:
Two experiments were conducted on two different datasets as called in this paper: Dataset A and Dataset B. Dataset A gave much better results but, it turned out that the dataset is highly erroneous and misleading. Therefore, data quality is of the utmost importance when training deep learning models, especially in the medical and health fields. The results of this study, show the ability of CNN and deep learning models in detecting the suspicious findings from the datasets. The DenseNet201 model not performing well on dataset B could be due to a variety of reasons such as the complexity of the ultrasound images or the relatively small number of data points available in the original dataset. To address this, the entire DenseNet201 model can be trained rather than freezing the feature extractor to perhaps produce better accuracy results on the test data of dataset B as training only the classifier seems to be not sufficient for this task. Also, experimenting with different learning rates and optimizers could yield more satisfactory results. However, the accuracy and reliability of the model’s predictions depend heavily on the quality of the data used to train it. If the data is flawed or biased, the model will likely produce inaccurate or unreliable results even if the results appear to be satisfactory. In the medical and health field, this can have serious consequences as it can lead to incorrect diagnoses or treatment recommendations, potentially causing harm to patients. Therefore, it is essential to ensure that the data used to train these models is of the highest quality and represents accurately the population intended to serve. This includes ensuring that the data is free from errors, and represents the target population, and has been collected using appropriate methods. Ensuring data quality is an ongoing process that requires continuous monitoring and improvement.
Journal:Syrian Journal for Science and Innovation
Abbreviation: SJSI
Publisher: Higher Commission for Scientific Research
Address of Publisher: Syria – Damascus – Seven Square
ISSN – Online: 2959-8591
Publishing Frequency: Quartal
Launched Year: 2023
This journal is licensed under a: Creative Commons Attribution 4.0 International License.